Exploring RDF Visualization Techniques for Enhanced Data Understanding
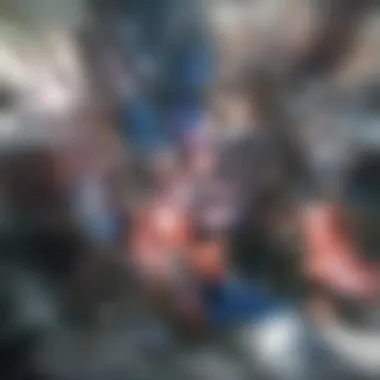
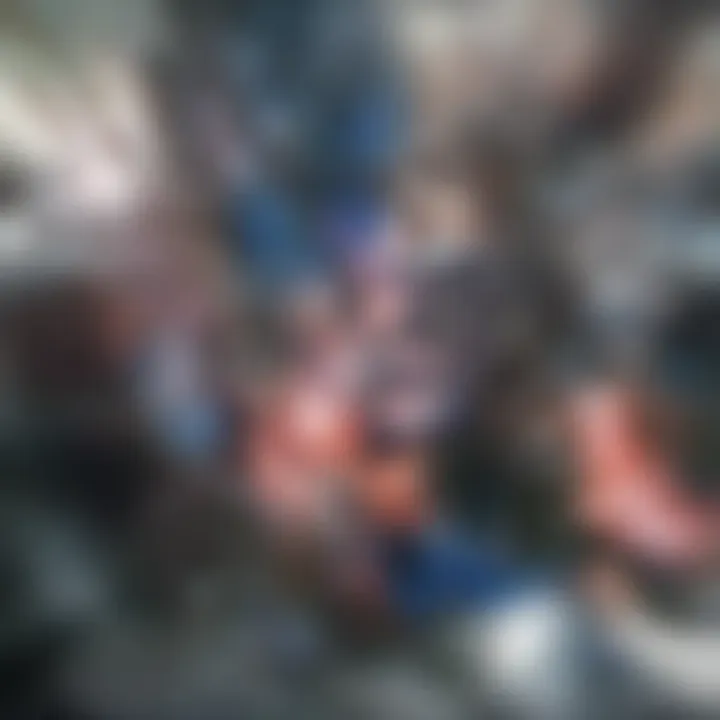
Intro
RDF (Resource Description Framework) is a critical component of the semantic web, serving as a foundational technology for representing information about resources in a structured way. Visualization of RDF data plays an important role in making this data more accessible and understandable. As datasets grow in complexity, the need for effective visualization techniques becomes paramount. Understanding the right tools and methodologies for RDF visualization can lead to better insights and enhance the usability of data across various sectors—including education, research, and industry.
In this article, we explore the significance of RDF visualization techniques and how they aid in the comprehension of data. We will dive into established practices, examine innovative tools available in the market, and discuss future trends. Our target audience includes students, educators, researchers, and professionals who seek to effectively interpret RDF datasets. This comprehensive guide will also highlight key concepts and terminology for better understanding.
Key Concepts and Terminology
Definition of Key Terms
RDF: The Resource Description Framework is a standard model for data interchange on the web, enabling the representation of information in a machine-readable format. RDF uses a subject-predicate-object structure to describe resources.
Visualization: In this context, visualization refers to graphical representations of RDF data. It helps in interpreting complex information and revealing connections that might not be immediately obvious.
Ontology: An ontology in RDF specifies the concepts and relationships within a particular domain, serving as a structured vocabulary that aids in data interpretation.
Concepts Explored in the Article
In this article, we cover various topics related to RDF visualization:
- The importance of RDF visualization in enhancing understanding.
- Techniques for visualizing RDF data, including various tools and methodologies.
- The evaluation of the effectiveness of different visualization approaches.
- Innovations in RDF visualization and their implications for the future.
Through these concepts, we aim to provide a well-rounded perspective on RDF visualization and its significance.
Findings and Discussion
Main Findings
As we explore RDF visualization techniques, several crucial findings emerge:
- Effectiveness of Visualization Tools: Tools like GraphDB, Protégé, and RDF4J provide users with different capabilities for visualizing RDF datasets. Each offers unique features catering to specific needs.
- Usability Issues: While many tools are available, usability remains a significant challenge. Some tools require a steep learning curve, limiting their adoption by less technical users.
- Integration with Other Data Types: There is an increasing trend towards integrating RDF visualization with other data types, enhancing the richness of data representation.
Potential Areas for Future Research
Future research can explore several promising areas:
- Advancements in Visualization Techniques: There is a need to develop new methods for visualizing larger and more complex RDF datasets.
- User-Centered Design: More emphasis on user experience design in RDF visualization tools can lead to better adoption and effectiveness.
- Interoperability: Improving the interoperability of RDF tools with other data systems can enhance data accessibility and usability.
Ultimately, effective RDF visualization is not just about creating appealing graphics; it is about ensuring that users can derive meaningful insights from complex datasets.
Prelude to RDF
The Resource Description Framework (RDF) has gained significance in recent years as the backbone of the semantic web. Understanding RDF is crucial because it lays the groundwork for how data is structured, shared, and connected online. In a world increasingly driven by data, RDF offers a standardized way to represent information, enabling better organization and retrieval.
RDF focuses on the relationships between data points, which is essential in allowing machines to interpret and understand the meaning behind the data, rather than just presenting it as raw information. This understanding is key for areas such as data integration, information sharing, and automated reasoning. The structured nature of RDF transforms the way we see and interact with data.
Another vital aspect of RDF revolves around its flexibility. It allows users to create data structures that can evolve over time without losing context or meaning. This adaptability directly addresses the modern challenge of handling vast volumes of information. Moreover, visualizing RDF data enhances its interpretability and usability, making it more accessible to a wider audience.
In this article, we will delve into the foundational elements of RDF, unpack its definition and purpose, and explore its historical context to set the stage for further exploration into visualization techniques. Understanding these initial concepts is essential for anyone looking to engage with RDF.
Definition and Purpose of RDF
RDF is a framework used for representing information about resources in a graph form. This graph consists of triples, which include a subject, predicate, and object. Each triple forms a statement about a resource, effectively linking pieces of data together. The primary purpose of RDF is to facilitate data interchange on the web, enabling different systems to understand and utilize information seamlessly.
RDF's versatility allows it to describe various data types, such as web pages, databases, or even physical objects. It supports complex relationships and can accommodate evolving data structures. This is especially important as organizations seek to manage interconnected and multi-faceted datasets.
Historical Context
The development of RDF began in the late 1990s, during the growth of the World Wide Web. The W3C (World Wide Web Consortium) introduced RDF as part of the effort to enhance web data interoperability. Early on, the lack of a standardized method for data representation was apparent, leading to confusion. Different platforms used varying data structures, hampering data sharing and communication.
The introduction of RDF aimed to resolve these issues by providing a common framework for data representation. Over the years, it has evolved alongside other technologies, such as SPARQL, which is used for querying RDF data. Today, RDF is a foundational element of semantic web technologies, supporting initiatives in various domains, including linked data, open data, and knowledge graphs.
In summary, RDF plays a pivotal role in structuring data in a way that is both machine-readable and human-comprehensible, paving the way for advanced exploration in visualization techniques.
Understanding Visualization
Visualization is an essential component when it comes to interpreting complex data sets, especially in the realm of RDF. The very nature of RDF involves intricate connections and relationships, which may overwhelm those unaccustomed to semantic web technologies. Thus, effective visualization serves not just as a representation of data but also as a bridge that closes the cognitive gap between raw data and human understanding.
Through visualization, users can gain insights much faster than by sifting through text-heavy information. Visual systems can quickly communicate patterns, trends, and outliers that might otherwise go unnoticed. Furthermore, different visualization techniques can cater to varied audiences, each with specific needs, enhancing overall engagement and understanding. It becomes more than an aesthetic component; it is foundational to the practical application of RDF in real-world scenarios.
The Role of Visualization in Data Interpretation
In the context of RDF, visualization plays a pivotal role in converting abstract concepts into tangible understanding. When data is presented visually, it becomes easier to identify relationships and hierarchies among entities. For instance, a node-edge diagram can succinctly illustrate how subjects are related to objects, demonstrating the interconnectivity of data points with utmost clarity.
Some of the key benefits of using visualization for RDF data include:
- Improved Clarity: Visualization simplifies complex information, allowing the audience to grasp it more easily.
- Enhanced Retention: People tend to remember visual information better than textual information.
- Quick Analysis: Visual mappings enable rapid identification of data correlations and anomalies, making analysis more straightforward.
By applying various visualization techniques, such as graphs or matrix layouts, users can distill relevant insights quickly, changing how data is consumed and understood. The ultimate goal is to facilitate decision-making based on clear, interpretable data.
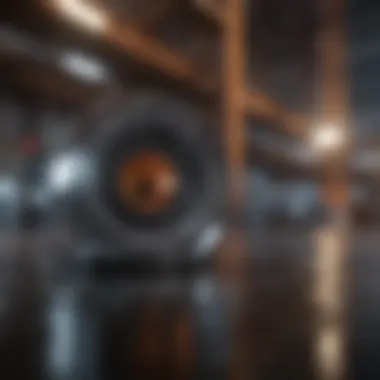
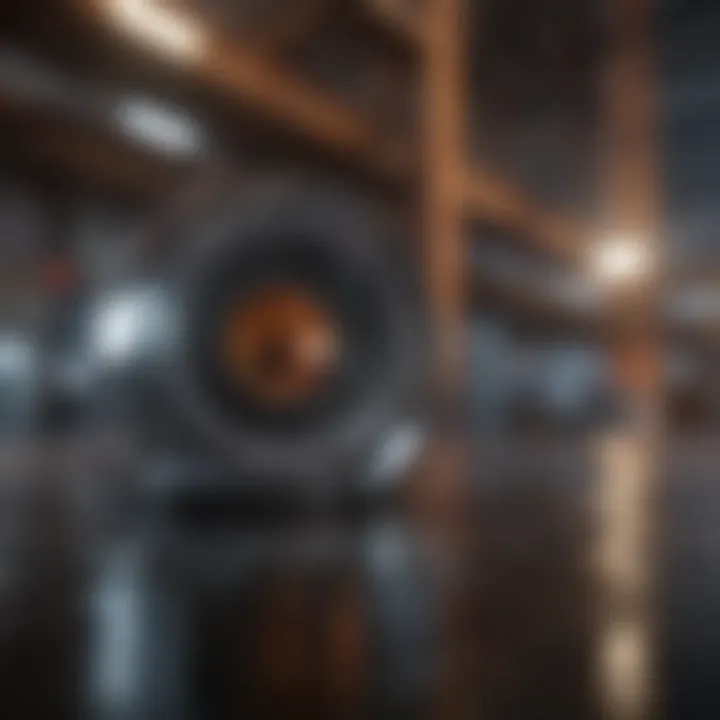
Cognitive Aspects of Visualization
Understanding the cognitive aspects of visualization is crucial for designing effective RDF representations. Humans tend to process visual information more efficiently than text. Our brains are wired to recognize shapes, colors, and spatial relationships which play a significant role in comprehension.
Some considerations in the design of RDF visualizations include:
- Gestalt Principles: Utilizing principles such as proximity, similarity, and closure can direct user attention and enhance understanding.
- Color and Contrast: Choosing appropriate color schemes aids in differentiating data sets and elevating the visibility of essential components.
- Layout and Organization: Effective arrangement of information supports the natural flow of data interpretation, making complex relations more manageable.
The cognitive aspect is tied closely to user engagement and interface design. A well-designed visualization can engage users cognitively, leading to better retention and comprehension of RDF datasets. As visualization techniques evolve, the focus must remain on making data interpretation intuitive and accessible.
RDF Data Structure
The RDF data structure is fundamental in the realm of semantic web technologies. Understanding how RDF organizes information is crucial for effective visualization of datasets. RDF, or Resource Description Framework, uses a specific data model that enables the representation of information in a way that is machine-readable, making it easier to interconnect and obtain insights from vast amounts of data.
Triple Structure of RDF
At the heart of RDF lies the triple structure. A triple consists of three components: subject, predicate, and object. This model allows RDF to describe relationships between resources in a simple and effective manner. Each triple can be seen as a statement about data where the subject denotes the entity, the predicate specifies the property or attribute, and the object indicates the value or another resource.
For example, consider a triple that states: "The Eiffel Tower (subject) is located in (predicate) Paris (object)." Such straightforward representations enable the formation of complex information networks. The usage of triples simplifies not only the understanding of relational data but also enhances the process of querying information via languages like SPARQL.
The benefits of this structure are significant. First, it provides clarity and consistency in data representation. Second, it allows for easy expansion. As new resources and relationships emerge, additional triples can be added without disrupting existing structures. This quality fosters a flexible data environment, which is essential for the dynamic nature of modern data applications.
Graphs and Their Representation
Graphs serve as a natural extension of the RDF triple structure. In this context, RDF triples can be mapped onto a graph where nodes represent subjects and objects while edges denote predicates. This transformation makes it possible to visualize complex interrelations at scale. Utilizing graphs for RDF data representation helps in identifying patterns, clusters, and relationships that may not be evident in traditional tabular formats.
Visualizing RDF data as graphs also enhances user comprehension. Stakeholders can quickly grasp data connections through visual cues rather than dense text or numbers. Furthermore, graph-based representations are particularly useful when dealing with large datasets. They allow for efficient navigation through immense data relationships, providing insights that could drive strategic decision-making in various domains.
"Graphs reveal the underlying structure of RDF data, enabling better understanding and interpretation, which is essential for data-driven strategies."
When considering tools for visualization, many software solutions offer graph-based representations that facilitate analytics and exploration of RDF data. These allow users to interact with the datasets dynamically, enhancing the overall experience in managing and interpreting RDF information.
In summary, the RDF data structure—comprising triples and graph representations—plays an integral role in the effective visualization of semantic web data. An understanding of these components is indispensable for extracting meaningful insights and fostering improvements across diverse fields.
Significance of RDF Visualization
RDF visualization is pivotal for translating complex relationships ingrained in RDF data into understandable formats. As the volume of data grows, the need for effective visualization techniques escalates. RDF's ability to represent knowledge as a graph facilitates diverse applications, but the underlying complexity often poses challenges in interpretation. Therefore, visualization techniques become essential tools for researchers, educators, and industry professionals aiming to derive insights from data.
Effective visualization of RDF data promotes clarity in representing relationships and hierarchies. Leveraging visual aids can transform abstract data into intuitive insights, enabling audiences to grasp intricate connections without wading through blocks of text. Furthermore, the significance of RDF visualization extends beyond mere representation; it influences decision-making and research trajectories by illuminating patterns that may not be immediately apparent through traditional analysis.
Facilitating Data Analysis
Visualizing RDF data supports analysts by simplifying data exploration. Complex datasets can be overwhelming and difficult to parse when viewed in tabular forms. Visual representations allow experts to identify trends, anomalies, and clusters efficiently. Analysts can intuitively engage with the data, simplifying the extraction of meaningful insights.
As a primary advantage, visualization promotes a deeper understanding of data relationships. For example, node-edge diagrams can showcase how resources relate within a dataset, revealing crucial links that traditional methods might obscure. Each connection tells a story, and skilled analysts can draw on these narratives to uncover additional layers of meaning.
This analytical approach promotes collaboration as well. When industry professionals or scholars share insights through visual methods, it can enhance collective comprehension. A shared visual context helps bridge gaps between different expertise areas, fostering cooperative data analysis efforts.
Improving Data Communication
Effective communication of RDF data is critical for stakeholders unfamiliar with its complexities. Visual representations provide a language that transcends technical jargon. By employing tools like tree structures or matrix representations, complex datasets can be distilled into coherent visual formats, providing clarity and engagement to a broader audience.
Communicating data effectively is essential for driving action. Enhanced visualization leads to improved data storytelling, which assists in various sectors, from academia to business. Well-designed graphs facilitate discussions and support strategic decisions, helping stakeholders understand significant findings without extraneous detail.
"Visualization is not just about seeing the data—it's about understanding it."
In summary, RDF visualization greatly enhances the ability to analyze and communicate data insights. By simplifying complexities and fostering a deeper understanding, RDF visualization aids both scholars and business professionals in making informed decisions. As visual tools advance, their integration into RDF analysis will likely continue to grow, emphasizing the vital role of visualization in the modern data landscape.
Common RDF Visualization Techniques
RDF visualization techniques are crucial for transforming abstract data into a more understandable format. They serve to translate the intricacies of RDF datasets into visual representations that facilitate analysis and communication. By employing these methods, researchers and professionals in the field of semantic web technologies can more efficiently interpret relationships within data.
Several techniques exist, each with distinct benefits and challenges. Understanding these methodologies is essential for anyone seeking to enhance data comprehension. This section delves deeper into three common RDF visualization techniques: node-edge diagrams, matrix representations, and tree structures.
Node-Edge Diagrams
Node-edge diagrams are one of the most recognized visualization methods for RDF datasets. In these diagrams, nodes represent entities, while edges illustrate the relationships between them. They are powerful for depicting connections visually, allowing viewers to quickly grasp complex interrelations.
Benefits:
- Clarity: These diagrams simplify the visualization of intricate connections, making them easier to interpret.
- Flexibility: They can be adapted to various datasets and can represent weighted relationships or different types of associations.
- Interactivity: Many tools allow users to interact with node-edge diagrams, enabling deeper exploration of data.
However, challenges exist. For vast datasets, node-edge diagrams can become cluttered, making it hard to discern meaningful patterns. Effective layouts and filtering options are essential for preventing information overload.
Matrix Representations
Matrix representations provide an alternative to node-edge diagrams. In this approach, both entities and relationships are presented in a grid, allowing users to see the connections at a glance. Each cell in the matrix indicates whether a relationship exists between the corresponding entities.
Benefits:
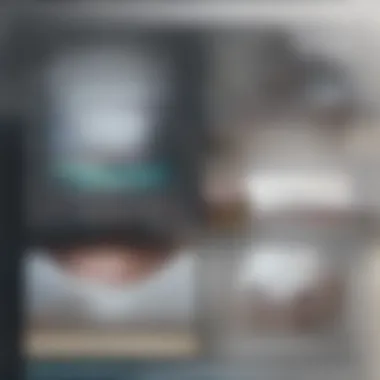
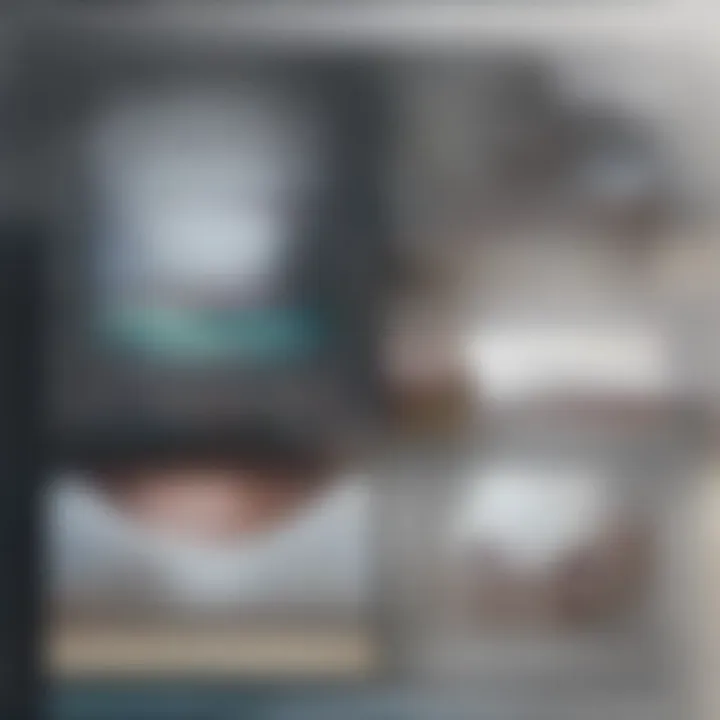
- Compactness: Great for summarizing large datasets in a systematic way.
- Efficiency: Quickly indicates which entities are related, without requiring extensive space.
However, this method may not visually convey the relationships as intuitively as node-edge diagrams. Sometimes, the sheer number of data points can lead to ambiguity. Understanding the underlying data structure is crucial for effective interpretation when using matrix representations.
Tree Structures
Tree structures organize RDF data hierarchically. In this visualization technique, parent nodes branch out to child nodes, clearly illustrating relationships. This method is particularly useful when representing ontologies or categorizing data hierarchically.
Benefits:
- Organization: They represent hierarchical relationships effectively, making them suitable for datasets with clear top-down structures.
- Simplicity: Users might find tree diagrams more straightforward for navigating complex relationships.
Like all methods, tree structures are not without drawbacks. They can oversimplify relationships, especially in datasets with interconnections among different branches. Users need to be cautious to ensure the tree does not distort the actual data relationships.
"Choosing the right visualization technique is fundamental for effective data communication in RDF."
Tools for RDF Visualization
RDF visualization plays a critical role in interpreting complex datasets, making the selection of the right tools essential. The tools available for RDF visualization can significantly influence how data is understood and utilized. They aid in simplifying intricate relationships represented in RDF, enhancing both analysis and presentation. With a diverse array of options, each tool offers unique features and capabilities, catering to various user needs and preferences. Selecting the appropriate tool can streamline the process of data interpretation and enhance the overall clarity of semantic connections.
Overview of Popular Tools
When discussing RDF visualization, several tools have gained recognition for their functionalities and ease of use. Some of the most notable tools include:
- Protege: Mainly used for ontology editing, it also includes plugins for visualizing RDF data, making it a versatile tool for semantic web projects.
- Gephi: This open-source software specializes in network visualization, allowing users to view RDF data as graphs. Its flexibility in handling large datasets adds to its popularity.
- Cytoscape: Initially designed for biological research, Cytoscape can be repurposed for RDF visualization. Its extensive functionality allows for rich interaction with data sets.
- Graphistry: Targeted for users who focus on visual analytics, it provides a platform to visualize complex graphs effectively.
- OntoGraf: A tool within the Protege framework, it enables users to visualize ontologies and RDF data interactively.
In addition to these, tools like D3.js and Vis.js allow developers to create custom visualizations, thus offering tailored solutions for specific needs.
Comparative Analysis of Tool Features
Understanding the features of each tool is crucial for determining their fit for specific projects. Several factors should be consideration when comparing RDF visualization tools. These include:
- User Interface: The user experience can dictate the efficiency of workflows. Tools like Protege and Gephi are known for their intuitive interfaces.
- Customization: Tools such as D3.js provide extensive options for visual customization, allowing for personalized visual presentations.
- Data Handling Capabilities: Some tools excel in managing massive datasets, while others might struggle with scalability. Gephi, for instance, is designed to handle large graphs efficiently.
- Interactivity: The level of interactivity offered by the tool can enhance user engagement. Cytoscape is known for its trend-setting features in this regard, especially in biological contexts.
- Integration: Compatibility with other tools or data formats can be essential for seamless workflows. Protege, for example, integrates well with other semantic web technologies.
"The choice of tool is directly influenced by the specific data visualization requirements and the user's comfort with the software."
In summary, the selection of RDF visualization tools is not merely a matter of preference; it crucially affects the interpretation of data. Understanding what each tool offers can empower users to make informed decisions that enhance the visualization process.
Challenges in RDF Visualization
RDF visualization presents unique challenges that are essential for understanding the effectiveness of graphical representations of data. These challenges are significant for enhancing the way users interpret, interact with, and draw conclusions from RDF datasets. The complexity of the data structure and the unique requirements of visualization present hurdles that must be addressed to improve usability and clarity.
Data Complexity
The inherent complexity of RDF data is a primary challenge in visualization. RDF datasets consist of interconnected triples that can represent vast amounts of information across different domains. Each triple includes a subject, predicate, and object, encapsulating relationships between various entities. This complexity can lead to convoluted visual representations that are difficult for users to comprehend.
To tackle data complexity, visualization tools need to employ effective strategies, such as:
- Simplification: Abstracting redundant or less important details to focus on critical relationships.
- Layered Visualization: Providing a multi-layered approach that allows users to drill down into specific details as needed.
- Custom Views: Allowing users to create personalized views that suit their particular interest or field of study.
These methods can significantly enhance the clarity of RDF visualizations, thereby making it easier to recognize patterns and relationships within the data.
Scalability Issues
Scalability is another crucial consideration in RDF visualization. As datasets grow in volume and complexity, the visualization tools must efficiently handle this increase without sacrificing performance or user experience. Poorly designed visual displays can become cluttered, making it hard to navigate large datasets.
To ensure scalability, developers should consider:
- Dynamic Loading: Implementing techniques that load data incrementally rather than all at once.
- Responsive Design: Creating visualizations that adapt to varying screen sizes and resolutions.
- Optimized Algorithms: Using algorithms that can manage large datasets efficiently without degrading performance.
By addressing scalability, visualization tools can support larger and more intricate datasets. This is essential as these resources become increasingly vital in research, industry, and education. In summary, overcoming challenges related to data complexity and scalability will lead to more effective and accessible RDF visualization, ensuring users can make informed decisions based on comprehensive data representations.
Best Practices in RDF Visualization
Visualizing RDF data is not merely about creating attractive graphics; it is about transforming dense and intricate datasets into understandable formats. Adhering to best practices in RDF visualization enhances clarity and engagement, which is essential for effective data communication. This section outlines key principles and strategies to optimize RDF visualizations, ensuring they are not only informative but also user-friendly.
Design Principles
Effective RDF visualizations rely on a few key design principles that enhance usability and interpretability. Clarity is paramount; each element should convey information without overwhelming the viewer. Here are some essential design principles:
- Simplicity: Aim for straightforward designs that prioritize essential data relationships. Avoid clutter to help users focus on what matters most.
- Consistent Layout: Use a consistent visual structure to aid recognition and comprehension. For example, maintaining uniform colors and shapes for similar data types can significantly improve understanding.
- Color Coding: A well-planned color scheme helps differentiate between categories of data and draws attention to critical components. Utilize color judiciously to prevent confusion.
- Hierarchy: Establish a visual hierarchy that guides users through the data. Important information should be more prominent, allowing users to grasp key insights quickly.
- Interactive Elements: Incorporate interactive features, like tooltips or zoom functionalities, to allow users to explore the data more deeply. This enables a more personalized experience.
By following these design principles, creators can ensure that their RDF visualizations facilitate understanding rather than complicate it. Successful implementation of these elements will ultimately lead to more effective communication of data insights.
User Engagement Strategies
User engagement is a critical aspect of RDF visualization. It is essential to employ strategies that attract and sustain the attention of users. In understanding the audience, one can tailor visualizations to meet their needs. Here are some effective strategies:
- Tailored Content: Know who your audience is and what they need from the visualization. Customize the content to resonate with specific user groups, whether they are researchers, students, or industry professionals.
- Guided Tutorials: Introduce users to the visualization with tutorials or walkthroughs. This can demystify complexities in the data and help users understand how to interact with the visualization.
- Feedback Mechanisms: Implement mechanisms for users to provide feedback on visualizations. This input can be invaluable for future improvements and adjustments.
- Community Engagement: Create a space for discussion around the visualizations. Platforms like Reddit or Facebook can serve as valuable forums for sharing insights and generating interest.
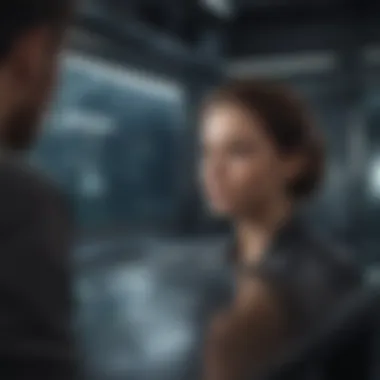
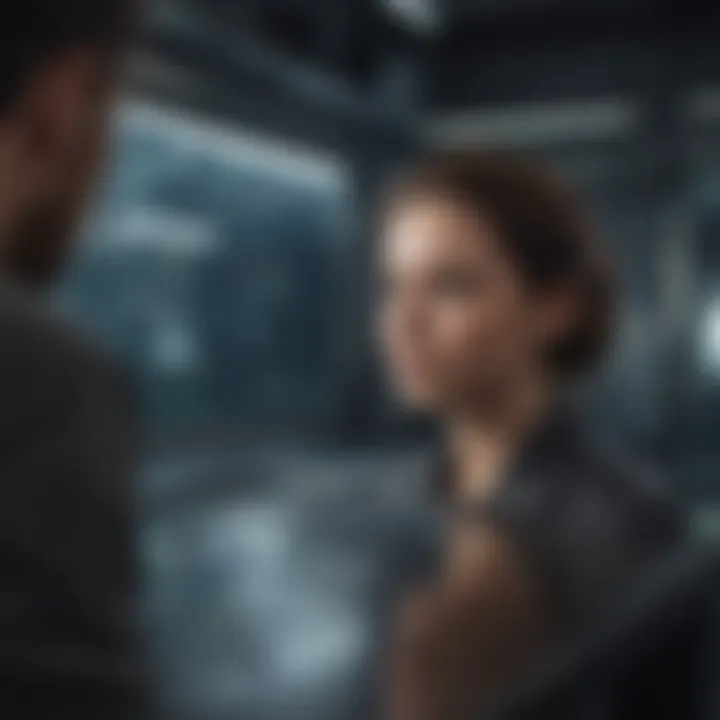
Employing these strategies can significantly improve user interaction with RDF visualizations, enhancing their overall experience. It is essential to create visualizations that not only display data but also invite exploration and understanding.
"Effective visualization is not just about art; it’s about science and communication."
By observing best practices in RDF visualization, one can transform complex data relationships into comprehensible and engaging formats. This ensures that users can derive insights efficiently, enhancing the utility of RDF as a framework for data representation.
Case Studies
Case studies are vital in understanding how RDF visualization techniques can be effectively engaged in real-world contexts. They allow researchers and professionals to observe the direct impact of these techniques on data comprehension and decision-making processes. Through specific examples, case studies can highlight successes and pinpoint challenges faced when implementing RDF visualizations. This examination informs best practices, guides future implementations, and enhances the overall understanding of semantic web technologies.
Successful Implementations in Academia
In academic settings, RDF visualization has proven to significantly enhance the learning experience. Universities and research institutions employ these techniques to represent complex datasets, enabling students and researchers to gain clearer insights. For example, the University of Cambridge developed a project utilizing RDF to map various academic publications. The visualization helped in identifying thematic connections between different papers, making knowledge discovery more efficient.
Another example comes from MIT, where researchers applied RDF visualization tools in analyzing large datasets related to machine learning. The students were able to interact with the graphical representation of data, leading to an improved understanding of how various algorithms influenced their applications.
Such implementations not only support data presentation but also encourage collaboration among diverse academic disciplines. Academic case studies present a practical model for using RDF visualization in structured learning environments, showcasing how it contributes to deeper insight and academic productivity.
Industry Applications
RDF visualization techniques are not only confined to academia; they extend their utility into the commercial sector as well. Industries like healthcare, finance, and information technology have started relying on these visual tools to manage and understand vast quantities of data.
For instance, a leading healthcare provider implemented RDF visualization to represent its patient data holistically. By visualizing relationships among patients, treatments, and outcomes, healthcare professionals improved their decision-making processes. This initiative led to increased patient satisfaction and operational efficiency.
Additionally, major firms in the finance sector such as Bank of America use RDF visualizations to navigate regulatory compliance and data governance challenges. By creating visual maps of complex regulatory requirements linked to customer data, they can enhance compliance strategies and minimize risks.
These cases illustrate the versatility of RDF visualization techniques beyond academia, highlighting their essential role in operational efficiency and strategic planning in various industries.
"The application of RDF visualization techniques can fundamentally transform how organizations perceive and manage their data, leading to more informed decisions."
Future Trends in RDF Visualization
The landscape of RDF visualization is evolving rapidly. It is crucial to understand the direction these changes may take. The future trends in RDF visualization embody significant elements that can enhance the comprehension and usability of complex data. They not only improve interaction but also expand the potential applications of RDF in various sectors.
Emerging Technologies
Emerging technologies play a pivotal role in shaping RDF visualization. Innovations in artificial intelligence and machine learning are being integrated into current visualization techniques, allowing for more dynamic interpretations of data. For example, AI can assist in identifying patterns within RDF datasets that may not be immediately apparent to human analysts.
Additionally, advancements in web technologies such as WebGL and interactive graphics are enhancing the visual representation of RDF graphs. Such technologies facilitate more immersive experiences in data exploration. By employing these tools, users can manipulate visual data in real-time, allowing for deeper insights.
New platforms, like Apache Jena and D3.js, offer tools tailored for RDF data. Their capabilities to transform graphs in a responsive and engaging manner highlight their importance in future use cases.
Potential Developments in User Interface Design
User interface design is another critical area where future trends are likely to emerge in RDF visualization. As users become more sophisticated, the demand for intuitive and user-friendly interfaces will rise. Simplicity in design will encourage wider adoption among non-technical users.
The implementation of personalized dashboards can make accessing and interpreting RDF data easier. Features such as drag-and-drop functionalities, customizable views, and interactive tutorials can empower users. This focus on accessibility is expected to bring RDF visualization to a broader audience.
Moreover, incorporating user feedback mechanisms will allow ongoing adjustments to interfaces based on what users find most effective. This will enhance not only user satisfaction but also the overall data exploration process.
"The intersection of technology and user-centered design can redefine how we visualize RDF, making it more accessible than ever."
In summary, the future of RDF visualization is promising. By embracing emerging technologies and prioritizing user interface design, we can expect a paradigm shift toward greater clarity and engagement with RDF data.
Closure
The conclusion of this article encapsulates the core themes and insights regarding RDF visualization techniques. It reinforces the notion that effective visualization is essential for comprehending complex RDF data sets. By visualizing these data structures, users can navigate intricate relationships and better understand the underlying semantics.
Summary of Findings
In summarizing the findings, it becomes clear that RDF visualization serves multiple purposes, including enhancing data analysis, improving communication, and facilitating knowledge discovery. Through various techniques such as node-edge diagrams, matrix representations, and tree structures, users can achieve a more intuitive grasp of RDF data. These visualization tools work in tandem to streamlining the process of data manipulation and interpretation.
- RDF visualization helps to uncover hidden patterns and insights within datasets.
- Different visualization techniques cater to distinct analytical needs and preferences.
- Effective tools are crucial in supporting these techniques, allowing users to explore RDF data dynamically.
Final Thoughts on the Importance of RDF Visualization
Final reflections highlight the increasing relevance of RDF visualization in the context of the expanding semantic web. As data grows more complex, the ability to visualize this information becomes increasingly critical. Understanding how to visualize RDF data not only supports improved analysis but also enhances collaboration across disciplines.
"A good visualization can break down barriers and foster understanding among diverse stakeholders."
Appendices
In the context of RDF visualization, the inclusion of appendices serves several important functions that enhance the overall understanding of the discussed topics. Appendices can be vital in providing additional resources, clarifying complex concepts, and offering supplementary information that complements the main body of the article. They provide a means to delve deeper into certain areas without disrupting the flow of discussion.
Specific Elements of Appendices
The appendices may include diagrams, code snippets, and extended examples of RDF visualization techniques. They can also comprise datasets used in case studies, which can serve as practical references for readers who wish to experiment with real-world data. By systematically organizing these materials, the appendices act as a repository of valuable information that readers can consult for clarification or further study.
Benefits of Including Appendices
- Enhanced Understanding: Appendices can help consolidate understanding by breaking down complex subjects into manageable parts.
- Resource Accessibility:
By providing links to resources such as datasets, relevant research papers, and software tools, they allow readers to explore topics more thoroughly. - Practical Application: Readers can find practical examples or templates that demonstrate the application of visualization techniques, fostering a hands-on approach to learning.
Considerations About Appendices
When crafting appendices, it is crucial to strike a balance between relevance and brevity. Only include materials that directly relate to the content discussed in the article. This focus ensures that the appendices remain useful rather than overwhelming. Additionally, clarity in organization helps readers navigate through the material efficiently.
In summary, appendices play a crucial role in enriching the discourse surrounding RDF visualization. They elevate the quality of the article by offering supplemental insight and practical aids that support a deeper exploration of the subject matter.