Grocery Demand Forecasting: Strategies and Insights
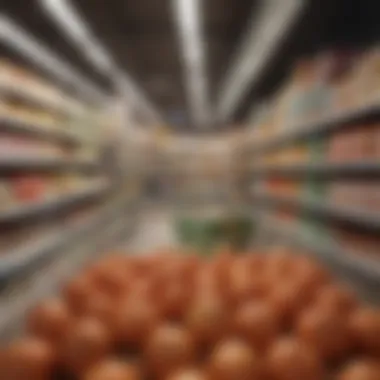
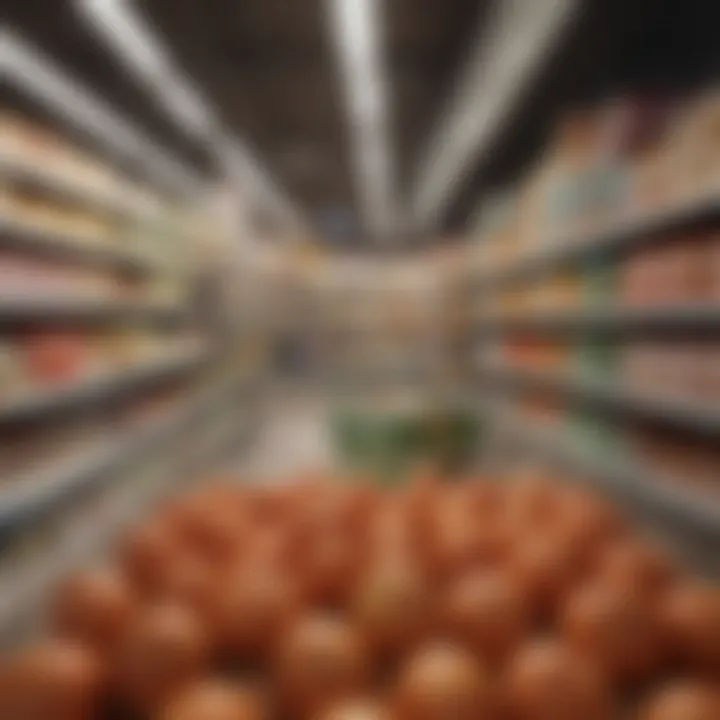
Intro
In the fast-paced world of grocery retail, understanding how to predict demand can mean the difference between thriving and just surviving. Consumers today expect not only variety but availability. Every visit to the supermarket can be a mixed bag; sometimes you find everything you need, while other times, items are out of stock or only half the shelves are filled. This inconsistency can be directly attributed to the effectiveness, or lack thereof, of grocery demand forecasting.
As supply chain management continues to evolve, the methods used to anticipate grocery demand have become more sophisticated. It's essential for industry players to grasp not only the statistical underpinnings of these methodologies but also the prevailing consumer behaviors, seasonality effects, and external factors that drive changes in demand. This article aims to shed light on these dynamics, ultimately equipping students, researchers, and industry veterans with the comprehension necessary for navigating the intricacies of grocery demand forecasting.
In what follows, we will discuss key concepts and terminology relevant to understanding forecasting methodologies, as well as highlight the main findings that emerge from this analysis.
Key Concepts and Terminology
Definition of Key Terms
To engage fully with the topic of grocery demand forecasting, it's necessary to define a few pivotal terms:
- Demand Forecasting: The process of estimating future demand for products or services, often using historical data and analytics.
- Supply Chain Management: The oversight and management of a network between a company and its suppliers, encompassing the movement of goods, information, and finances.
- Seasonality: The tendency for demand to rise and fall at particular times of the year, influenced by factors such as holidays, seasonal produce availability, and weather patterns.
- Consumer Behavior: The study of how individuals make decisions to spend their available resources, including time and money, on consumption-related items.
Concepts Explored in the Article
Several essential concepts underpin grocery demand forecasting, which will be elaborated on in the subsequent sections:
- The role of advanced analytics and big data in shaping forecasting practices.
- Traditional statistical methods versus modern analytical techniques.
- The influence of consumer trends and behaviors on forecasting accuracy.
- External factors such as economic changes, health crises, and natural disasters that disrupt established trends.
Findings and Discussion
Main Findings
Grocery demand forecasting presents a complex landscape of challenges and opportunities. Some key findings from this exploration include:
- Technological Integration: Organizations adopting tools like machine learning and artificial intelligence significantly enhance their forecasting accuracy.
- Consumer Insights: Understanding consumer purchasing patterns can improve inventory turnover and reduce waste.
- Impact of Seasonality: Recognizing seasonal trends can aid in planning inventory levels and promotional activities, ensuring optimal stock levels during peak times.
- Real-time data processing and predictive analytics can adjust supply chain strategies dynamically.
- Practices like segmenting customers by demographics help tailor inventory more precisely to demand segments.
"Accurate forecasting isnât just about numbers; itâs about understanding people and the world around them."
Potential Areas for Future Research
The field of grocery demand forecasting is constantly evolving, opening doors for further research. Here are a few potential areas to explore:
- The effects of global events, such as pandemics, on grocery shopping behavior and demands.
- The long-term impacts of sustainability trends on consumer purchasing decisions.
- Further integration of emerging technologies in supply chain management processes.
By diving into these themes, we can gain a clearer, more nuanced understanding of grocery demand forecasting and its significance to operational efficiency and customer satisfaction.
Prelude to Grocery Demand Forecasting
In the fast-paced world of grocery retail, understanding what consumers will buy and when they will buy it is paramount. Grocery demand forecasting serves as the backbone of supply chain management, ultimately influencing sales, inventory control, and customer satisfaction. This isnât just a matter of stocking shelves but rather a sophisticated dance that involves predicting consumer behavior and aligning it with available resources. The need for accurate forecasting has never been more apparent than in the last few years, where consumers displayed behaviors that were perhaps unpredictable.
From fresh produce to packaged goods, every item has its own demand rhythm. Grocery demand forecasting therefore acts as a compassâguiding retailers through uncharted waters, especially during peak seasons and unpredictable market shifts. In essence, it allows businesses to not only stay afloat but to thrive during the uncertainties presented by changing consumer trends or external disturbances, like economic crises or pandemics.
Importance in the Supply Chain
The role that demand forecasting plays within the supply chain cannot be overstated. Accurate predictions allow retailers to maintain the delicate balance between supply and demand. When a retailer can accurately predict what will be needed, it helps in optimizing inventory levels, thereby reducing waste. For instance, if a store knows that demand for organic bananas tends to spike every summer, they can order precisely what they need instead of overstocking and facing potential spoilage. On the flip side, missing these cues could lead to empty shelves, frustrating customers and causing them to seek alternatives elsewhere.
Moreover, effective demand forecasting bolsters supplier relationships. By knowing the needs in advance, grocery stores can communicate clearly with suppliers about what and when they need. This strengthens supply chain dynamics, ensuring that both suppliers and retailers can work efficiently together. Consequently, the whole supply chain benefits from less overproduction and better resource allocation.
Historical Context
Delving into the historical context of grocery demand forecasting opens a window into how far the industry has come. In earlier times, forecasting primarily relied on gut feelings, historical sales data, and sometimes, sheer guesswork. Retailers would observe patterns, like increased snack sales during back-to-school seasons but lacked sophisticated measurement tools to understand these trends deeply.
With technological advancements in the twentieth century, particularly the rise of computers and statistical analysis, the landscape shifted dramatically. Simple spreadsheets gave way to complex algorithms, allowing for more precise and data-driven demand forecasts. In retrospect, the relationship between technology and grocery demand forecasting developed hand-in-hand, pushing the boundaries of whatâs possible. Today, the evolution continues with machine learning and big data playing an integral part in painting a clearer picture of consumer behaviorâeach step informing future strategies.
As we navigate the ins and outs of grocery demand forecasting in subsequent sections, it is essential to appreciate its significance not just as a tool for efficiency, but also as a critical element shaping the retail landscape. Understanding its roots gives us a profound perspective on its present and likely future developments.
Key Concepts in Demand Forecasting
Grocery demand forecasting stands as a cornerstone for the food industry, intertwining various elements that influence operational success. In this section, we will dissect the fundamental concepts that underpin accurate demand forecasting, emphasizing the essential terms and patterns that are crucial for industry stakeholders.
Definitions and Terminology
Understanding the core terminology is pivotal in navigating the complex landscape of grocery demand forecasting. Let's break down some key terms that are frequently encountered:
- Forecast: It refers to an estimate or prediction of future demand, often based on historical data and trends.
- Demand Function: The relationship that shows how the quantity of a product demanded is affected by factors like price, consumer preferences, and income levels.
- Time Series Analysis: A statistical technique that analyzes data points collected or recorded at specific time intervals; crucial for understanding trends over time.
- Lead Time: The amount of time between ordering and receiving goods, which plays a significant role in inventory management and stock replenishment.
- Error Metrics: Tools to measure the accuracy of forecasts, such as Mean Absolute Error (MAE) and Root Mean Square Error (RMSE).
Understanding these definitions provides a framework for making informed decisions and strategies that align with market demands.
Demand Variability and Patterns
Demand for grocery items is rarely static. Fluctuations happen for a myriad of reasons, and recognizing these patterns can significantly aid in forecasting efforts. Here are a few points to consider:
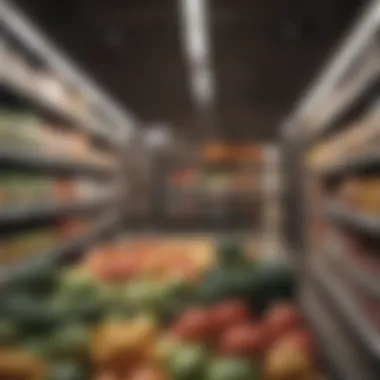
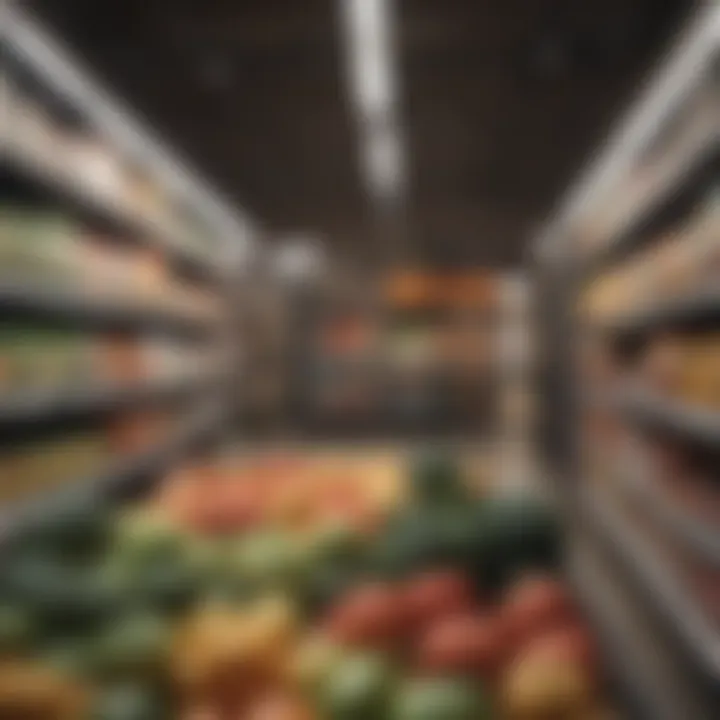
- Seasonality: Certain goods see spikes in demand during specific times of the year. For example, ice cream sales soar in summer while turkey consumption peaks around Thanksgiving.
- Trends: Launches of new health studies or social media influences can shift consumer preferences, causing unexpected surges or drops in demand.
- Randomness: Not every fluctuation can be predicted. Unforeseen events, such as natural disasters or public health emergencies, can lead to erratic demand that challenges forecasting models.
Identifying these demand patterns requires continuous monitoring and analysis. The right tools and methodologies enable businesses to adapt quickly to changes.
Traditional Forecasting Methods
In the realm of grocery demand forecasting, traditional forecasting methods have proven essential. These methods, steeped in a rich history of statistical analysis, provide a fundamental approach to predicting consumer needs and preferences. Understanding these methods is important because they deliver a baseline for comparison against modern techniques. The strengths and weaknesses of traditional approaches can assist in honing the application of cutting-edge technology, ensuring that businesses navigate the complexities of grocery demand successfully.
Qualitative Approaches
Qualitative approaches to forecasting are inherently subjective but can yield insightful data on consumer behavior. Techniques such as expert judgment and focus groups capture the nuances of market sentiment, providing context that raw data often lacks. For instance, consider a grocery store trying to decide how much organic produce to stock. Through discussions with regular customers, the manager might learn that there's a growing interest in organic diets fueled by social media trends, thus influencing ordering decisions.
Key characteristics of qualitative methods include:
- Subjectivity: They rely on human judgment, meaning results can vary.
- Rich Insights: They can expose underlying drivers of consumer preferences.
- Flexibility: Adaptable to changing conditions and trends.
However, these methods might be less reliable than quantitative ones due to potential biases.
Quantitative Techniques
Quantitative techniques embrace numerical data and statistical analysis, making them immensely popular for forecasting grocery demand. They offer accuracy and replicability, traits that make them preferable for many businesses.
Moving Averages
Moving Averages smooth out fluctuations in data to reveal trends over time. Theyâre particularly helpful in identifying demand patterns that can influence stock levels. The trend line these averages create allows retailers to make more informed decisions. A notable characteristic of moving averages is their ability to account for seasonal variations; each period's average informs the next. This straightforward approach is appealing due to its simplicity, yet it carries the inherent risk of lagging indicators.
- Advantages: Easy to calculate and understand, ideal for stable demand.
- Disadvantages: May overlook sudden changes in consumer behavior.
Exponential Smoothing
Exponential Smoothing takes Moving Averages a step further by weighing recent observations more heavily than older ones. This approach is effective for grocery stores facing quickly changing demand, allowing them to respond to trends faster. Its main characteristic is the smoothing constant, which dictates the weight of data from the past. A store may benefit from a higher weight on recent sales data during holiday seasons when demand surges.
- Advantages: Quick to adapt to recent data changes, reduces lag.
- Disadvantages: Can be overly sensitive to sudden market shocks.
Regression Analysis
Regression Analysis focuses on understanding the relationship between various factors and demand. For example, a grocery chain might analyze the impact of promotional events on sales to predict future outcomes accurately. A key characteristic is its ability to incorporate multiple variables, presenting a more comprehensive view of demand influences. The unique feature here is its versatility â it allows for various forms of analysis from simple linear to more complex models.
- Advantages: Captures relationships in a wide data range, highly customizable.
- Disadvantages: Requires a robust dataset and can be complex to implement.
Understanding these traditional forecasting methods not only lays the groundwork for developing future strategies but also enhances a grocery storeâs overall efficiency. As the market continues to evolve, blending these techniques with modern approaches will be essential for staying ahead of the curve.
Modern Analytical Techniques
The landscape of grocery demand forecasting continues to evolve, notably due to remarkable analytical advancements that transform the way businesses predict consumer behavior and stock requirements. Modern analytical techniques leverage technology, allowing grocery retailers to harness vast amounts of data to gain actionable insights. These techniques not only improve accuracy in predictions but also streamline operations across the supply chain. As consumer dynamics shift rapidly, traditional methods may fall short, making it crucial to embrace innovative approaches.
Machine Learning Applications
Machine learning applications fall under the umbrella of modern analytical techniques, signifying a transition from simple data analysis to more complex algorithmic processing. This method enables the development of predictive models that can learn from past data and enhance forecasting over time.
Supervised Learning Models
Supervised learning models refer to algorithms that learn from labeled datasets. The model is trained on a portion of data where the outcome is known. Then, it's tested against another dataset to measure predictive performance. This approach is particularly beneficial for demand forecasting, as it captures historical patterns in sales data.
One key characteristic of supervised learning is its reliance on defined outcomes, which provides a structured way to interpret data. A beneficial aspect is the ability to identify trends, like seasonal sales spikes, illuminating patterns that can aid stock replenishment. However, one disadvantage is that it requires a substantial amount of high-quality labeled data to be effective, which might not always be readily available.
"The true value of supervised learning lies in its capacity to unveil hidden relationships among various variables that influence demand."
Unsupervised Learning Models
Unsupervised learning models, on the other hand, explore unlabeled data to find inherent patterns or groupings. This method is particularly useful in identifying market segments or atypical consumer behavior that may not be apparent through traditional analysis methods.
One distinctive feature of unsupervised learning is its ability to process large datasets without the prior definition of outcomes, allowing businesses to uncover new insights. This characteristic makes it popular as it helps in discerning natural clusters or trends. Nonetheless, it may pose challenges when it comes to interpreting the results since the model does not provide explicit labels or outcomes.
Big Data Analytics
Moving beyond machine learning, big data analytics plays a crucial role in modern analytical techniques. The sheer volume, variety, and velocity of data generated in todayâs digital age necessitates robust analytical frameworks capable of processing vast datasets in real-time. This practice enhances the granularity of forecasting, providing insights that were previously overlooked.
Benefits of big data analytics for grocery demand forecasting include:
- The ability to analyze consumer buying habits based on real-time data.
- Better responsiveness to changes in market dynamics.
- Improved inventory management through accurate predictions driven by analytics.
The integration of big data analytics with machine learning methodologies can further enhance predictive capabilities, leading to more refined forecast models. This combination forms a powerful approach to decipher and anticipate grocery demand in an ever-changing retail environment.
The Role of Technology in Forecasting
The landscape of grocery demand forecasting has transformed dramatically due to the rapid advancement in technology. In todayâs fast-paced market, relying on intuition or outdated methods can be a recipe for disaster. Instead, businesses are utilizing cutting-edge tools and technologies to improve accuracy in demand prediction. The role of technology in forecasting not only streamlines operations but also enhances responsiveness to market fluctuations. Furthermore, it empowers grocery retailers to meet customer needs more effectively and keeps inventory levels optimized.
Software Solutions and Tools
A plethora of software solutions available today cater to various aspects of demand forecasting. These applications leverage algorithms and data analytics to provide insights that were previously unattainable with conventional methods. Letâs break down some significant tools and their benefits:
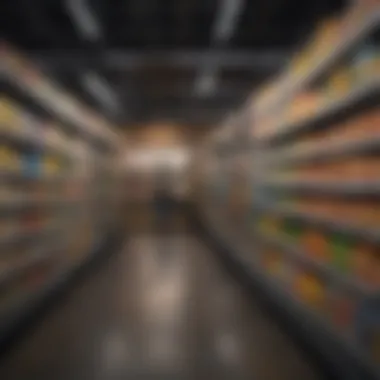
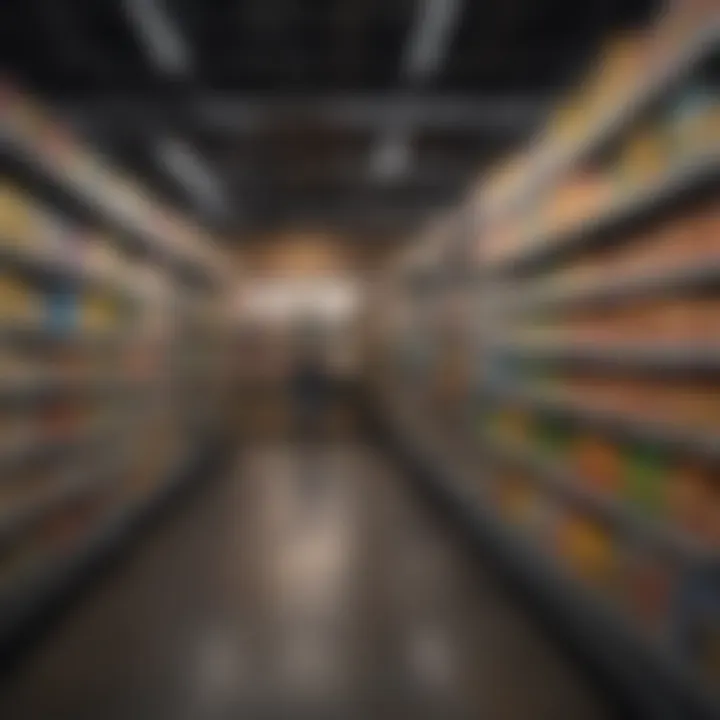
- Forecasting Software: Platforms like SAP Integrated Business Planning and Oracle Demantra offer comprehensive solutions for demand planning. These tools utilize historical data coupled with machine learning to generate accurate forecasts.
- Business Intelligence Tools: Applications such as Tableau and Power BI synthesize data from various sources. They visualize key metrics and patterns, allowing decision-makers to see the bigger picture more easily.
- Inventory Management Systems: Tools like Fishbowl or NetSuite automate restocking processes and keep track of inventory in real-time. This helps in avoiding out-of-stock scenarios and overstocking, contributing to better cash flow.
These technologies do not merely automate processes; they provide a safety net for businesses to make informed decisions. By analyzing vast amounts of consumer data, these tools uncover trends that might otherwise go unnoticed.
Data Integration and Management
Data integration is the backbone of effective grocery demand forecasting. In a world inundated with information, managing this data is crucial. Retailers often collect data from various sourcesâpoint-of-sale systems, customer feedback, market surveys, and social media. Here are some considerations for effective data management:
- Unified Data Platforms: Using platforms that aggregate data from multiple sources ensures that everyone in the organization works with the same accurate information. Tools like Microsoft Azure or AWS Redshift provide such data warehouses.
- Data Quality Assurance: Itâs essential to scrutinize the accuracy of the data being fed into forecasting models. Poor-quality data can lead to disastrous forecast inaccuracies, resulting in financial losses. Regular audits and cleansing processes help maintain integrity.
- Real-Time Data Access: Faster decision-making relies on real-time access to data. Implementing systems that allow instantaneous updates can keep businesses agile and ready to adapt to changes.
âData is the oil of the 21st century, and analytics is the combustion engine.â
â Peter Sondergaard
External Influences on Demand
Understanding external influences on demand is critical for accurate grocery demand forecasting. These influences shape customer purchasing behavior, directly affecting supply chain dynamics. From shifts in market trends to the impact of special events, these factors cannot be overlooked as they contribute significantly to forecasting models. Incorporating these elements not only enhances the reliability of predictions but also aligns operational strategies with actual consumer needs.
Market Trends
Market trends serve as the backbone of understanding consumer preferences. They reflect evolving behaviors and attitudes towards various products. For instance, an increased awareness of health issues has led consumers to seek organic and whole food options, dragging grocery chains to rethink product placements and inventories.
Some key elements influencing market trends include:
- Consumer Insights: Surveys and focus groups can reveal preferences that larger datasets might miss.
- Social Media Buzz: Platforms like Reddit and Facebook provide real-time insights into what people are talking about and purchasing.
- Competitive Landscape: Keeping tabs on what competitors are offering can provide a benchmark of what the demand unearths.
A savvy business must not only listen to sales data but also tune into the cultural zeitgeist that actively shapes those numbers.
Seasonality and Economic Factors
Seasonality is another beast, and its influence is undeniable. Grocers often see spikes in demand for certain products during particular seasons. For instance, creamy ice cream sales often soar during summer months while hearty soups find their sweet spot in winter. Consequently, understanding seasonality allows for better inventory management.
On the economic front, factors such as inflation or unemployment rates can drastically shift consumer spending habits. When the economy is thriving, people are more likely to splurge on premium items. However, in tougher times, budget-friendly options dominate the shopping carts. Analysts often utilize historical data to assess seasonal trends and forecast accordingly.
Impact of Events and Holidays
Holidays and special events also have strong implications on grocery demand forecasting. For example, the days leading up to Thanksgiving are notorious for bulging shopping lists, with turkey sales soaring. Conversely, during quieter periods, sales can wane significantly.
Understanding these annual shifts is important not only for stock management but also for marketing campaigns aimed at consumers. Promotions around specific holidaysâlike discounts for Valentineâs Day chocolates or grilling essentials for the Fourth of Julyâcan effectively boost sales.
When grocery chains align their inventory with consumer expectations during these times, they can drive foot traffic and virtual visits, ultimately enhancing revenue.
In summary, recognizing and adapting to external influences is paramount. Keeping a finger on the pulse of market trends, understanding seasonal fluctuations, and leveraging the power of events allows grocery businesses to stay ahead of the curve and achieve operational excellence. Effective demand forecasting is indeed a balancing act of anticipation and observation.
Challenges in Grocery Demand Forecasting
Understanding the challenges in grocery demand forecasting is fundamental for professionals seeking to enhance efficiency in the retail food sector. The complexities in this area often arise from the interplay of various factors, which makes accurate forecasting a daunting task. When these challenges are not adequately addressed, they could lead to significant consequences ranging from excess inventory to missed sales opportunities. It is essential to dive into the reasons why demand forecasting can be tricky, and what implications this holds for grocery retailers.
Data Quality Issues
One of the primary hurdles in grocery demand forecasting is the quality of data used for making predictions. Poor quality data can originate from multiple sourcesâfrom flawed point-of-sale systems to incomplete inventory records. If the data fed into analytics tools is inaccurate, heavily biased, or inconsistent, the resulting forecasts will inevitably suffer.
- Examples of data quality issues include:
- Inconsistent coding of products, which leads to misunderstandings.
- Missing historical data from previous sales trends, which are vital for establishing patterns.
- Human error in data entry or reporting, potentially skewing overall results.
The existence of these issues can lead to a âgarbage in, garbage outâ scenario, where the insights gained from the data can mislead strategic decision-making. Addressing data quality should be central to a robust forecasting strategy, encompassing regular audits, data cleaning processes, and validation checks on data inputs.
Consumer Behavior Dynamics
Consumer behavior is another variable that can complicate grocery demand forecasting. Shifts in consumer preferences, driven by trends, marketing influences, or socio-economic factors, can create sudden spikes or drops in demand that traditional models may fail to capture.
- For instance:
- The rising trend of plant-based diets can cause substantial increases in demand for related products.
- Seasonal events such as holidays can alter purchasing patterns dramatically, causing unexpected trends.
Understanding why consumers make specific choices at particular times can be a challenge, yet itâs crucial for predictions. Retailers are advised to invest into analytics tools that assess consumer sentiment and behavior, not just historical buying patterns. Predicting shifting behaviors requires monitoring social media, customer feedback, and emerging trendsâessentially, having a finger on the pulse of the market.
Integration of Diverse Data Sources
The integration of diverse data sources poses yet another challenge in achieving reliable forecasts. In todayâs digital age, grocery retailers have access to a myriad of data types, including online sales, customer loyalty programs, and supply chain logistics. However, pulling together these varied data streams into a coherent picture can be a laborious task.
- Key considerations include:
- How do we combine data from multiple channels for a comprehensive view of demand?
- Are we considering external factors such as economic indicators or demographic changes?
- What tools are in place to ensure that data from all sources is synthesised effectively?
These questions underscore the importance of having proper integration tools and methodologies. Leveraging advanced technologies like cloud-based data warehousing can help simplify these integrations. Ultimately, a more centralized approach can offer better insights that inform more effective forecasting models.
"In forecasting, the ability to aggregate and interpret diverse data seamlessly can make or break the strategy."
Together, these challenges form a complex landscape that grocery retailers must navigate to remain competitive. As consumer expectations evolve and market conditions change, continuously refining demand forecasting processes will be pivotal for sustaining growth.
Best Practices for Forecasting

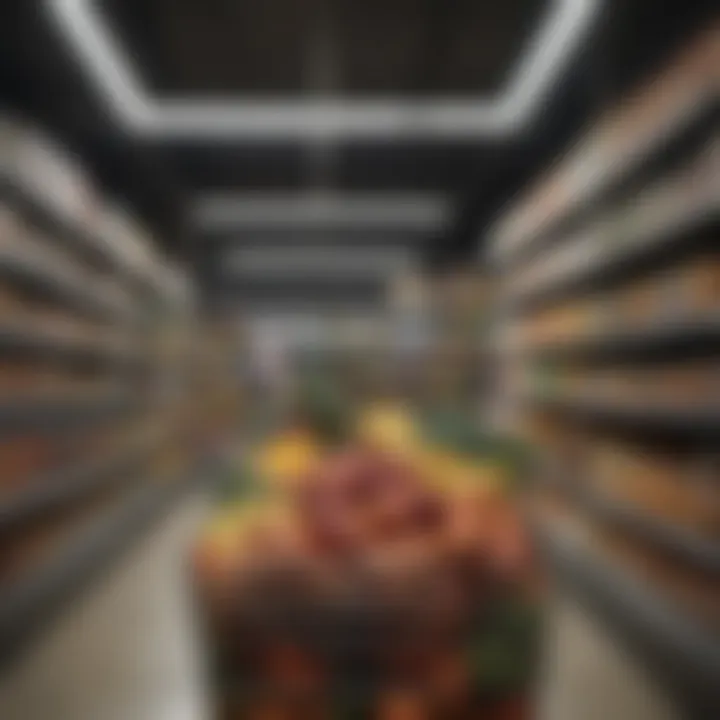
Effective grocery demand forecasting is not a one-size-fits-all approach; it requires a nuanced understanding of various operational facets. The goal here is to not just predict numbers, but to strategically align those predictions with business objectives. When we think about the best practices in this area, two aspects stand out: collaboration across departments and continuous improvement processes. Both play a crucial role in refining forecasting accuracy and enhancing company responsiveness.
Collaboration Across Departments
Collaboration among different departments is more than just working in silos. It's about integrating knowledge and expertise from diverse areas such as sales, marketing, inventory management, and even finance. When these teams come together, they bring unique perspectives that can significantly enhance the accuracy of demand forecasting.
- Sales Insights: The sales team has a finger on the pulse of customer preferences and buying habits. They can provide feedback on promotional activities and their immediate impact on demand, which is invaluable data that can fine-tune forecasts.
- Marketing Input: Marketing teams understand seasonal trends and can predict spikes in demand due to campaigns or new product launches. They see the bigger picture and their input is crucial for anticipatory shifts in consumer behavior.
- Inventory Coordination: Inventory managers contribute insights into stock levels and the implications for ordering frequencies. Their input can help closely align forecasts with what is feasible operationally.
The symbiotic relationship among these departments ensures that all angles are considered when making predictions. When teams share data and insights freely, it translates to a more accurate and robust forecasting model. Without this level of collaboration, forecasts can quickly go awry, leading to stockouts or excess inventory.
Effective forecasting is a team sport. - Successful demand forecasting relies heavily on effective communication and collaboration between all departments.
Continuous Improvement Processes
The landscape of grocery demand forecasting is constantly evolving due to new technologies and shifting consumer behaviors. Establishing a framework for continuous improvement processes is therefore essential for organizations aiming to stay ahead.
- Data Review: Regular reviews of forecasting models are imperative. This involves analyzing past forecasts against actual sales to identify gaps and discrepancies. Adjusting models based on real-world outcomes helps refine future predictions.
- Feedback Loops: Creating feedback loops is an effective method for ongoing improvement. This might involve regular meetings where teams assess what worked and what didnât in previous forecasting attempts. The continuous sharing of this information ensures a dynamic approach to refinement.
- Training and Development: Investing in training ensures that the forecasting team is well-versed in the latest methodologies and tools. As new techniques emerge, having knowledgeable staff allows organizations to adopt them effectively.
The emphasis on continuous improvement ensures that organizations are not just resting on their laurels. Instead, they actively seek ways to enhance their forecasting processes and adjust to new challenges as they arise.
Case Studies in Forecasting Success
In the realm of grocery demand forecasting, real-world applications and proven successes stand as powerful testaments to the efficacy of various methodologies. By examining case studies, one can glean valuable insights that illuminate the path forward for both established retailers and new entrants in the market. These examples not only showcase the techniques used but also highlight both the benefits and the critical considerations tied to successful demand forecasting.
Retail Giants
Consider the approach taken by Walmart, a titan in the grocery industry. Walmart employs a sophisticated forecasting model that integrates sales data, local market trends, and even real-time weather information to adjust inventory levels dynamically. For instance, during hurricane season, Walmart has been known to ramp up its stock of bottled water and non-perishable foods in areas that are likely to be affected. This proactive approach not only maximizes their sales during these critical times but also ensures customer satisfaction through reliable product availability.
- Key Elements to Note:
- Data-Driven Decision Making: Walmart's forecasting model aligns closely with its vast data analytics, enabling it to anticipate customer needs.
- Flexibility: The ability to adjust forecasts in real-time based on external factors is crucial for success.
Another example is Tesco, a British retail giant known for utilizing demand forecasting software to manage its extensive supply chain. Tescoâs system analyzes historical sales data and combines it with current consumer behavior trends, ensuring optimized stock levels across various products. When launching seasonal items, such as summer barbecue essentials, Tesco adjusts inventory according to forecasts derived from past performance, social media trends, and even regional events.
- Benefits:
- Improved Inventory Management: Reduces waste and out-of-stock instances.
- Enhanced Customer Experience: Products are available when consumers need them, boosting loyalty and satisfaction.
Innovative Startups
Turning to the other end of the spectrum, we find startups that are breaking the mold with cutting-edge forecasting techniques. Take, for example, Instacart, an online grocery delivery service that relies heavily on predictive analytics. Instacart gathers data from multiple customers as they interact with the platform, allowing the company to refine its demand forecasts over time.
- Unique Insights from Instacart:
- User Behavior Analysis: Understanding how consumers modify their shopping lists or respond to prompts can yield essential data for forecasting.
- Agile Adaptation: Startups often pivot quickly based on real-time feedback, making them adept at handling uncertainty in demand.
Another noteworthy startup is Boxed, an online wholesaler targeting bulk purchasing for consumers. Boxed uses innovative analytics tools to track purchasing patterns and seasonality trends effectively. For instance, around back-to-school season, they ramp up inventory for essential school supplies based on previous yearsâ data.
- Considerations for Success:
- Investment in Technology: Innovative startups often rely on advanced analytics software, machine learning, and AI to outmaneuver traditional players.
- Niche Marketing: Tailoring products to specific consumer segments can yield high returns on investment in a competitive market.
In summary, understanding how different playersâwhether they are industry giants or nimble startupsâapproach grocery demand forecasting provides vital lessons. The detailed scrutiny of these case studies reveals that integrating technology with a solid grasp of market dynamics is paramount for any player aiming to thrive in the complex landscape of grocery retail.
Future Trends in Demand Forecasting
The landscape of grocery demand forecasting is evolving, and understanding future trends in this area is crucial for organizations aiming to stay ahead of the competition. As technology advances and consumer behaviors shift, the methods used to predict grocery demand are becoming increasingly sophisticated. Artificial Intelligence and predictive analytics are at the forefront of these developments, offering numerous benefits to businesses in anticipating customer needs, optimizing inventory, and improving overall operational efficiency.
Artificial Intelligence Integration
The adoption of Artificial Intelligence (AI) in grocery demand forecasting holds the potential to revolutionize the way businesses approach data analysis. By harnessing the vast amounts of data generated daily, AI can identify patterns and trends that may not be apparent through traditional methods. Machine learning algorithms can digest numerous variables, including seasonal trends, local events, and even social media sentiment, providing a more nuanced forecast that considers diverse influences on consumer purchasing behavior.
For instance, consider a grocery store that utilizes AI to analyze buying patterns over several years. The software might decide, based on historical sales data, that sales of fresh produce improve significantly when a local sports team wins a game. With this data, managers can then prepare for potential spikes in related purchases post-game, leading to improved stock levels and reduced waste.
"AI allows businesses to not just guess, but to know what customers are likely to buy next. Itâs like having a crystal ball thatâs fueled by data rather than magic."
Predictive Analytics Advancements
Predictive analytics has seen significant advancements recently, driven primarily by improvements in data processing technologies and deeper analytical capabilities. This discipline focuses on analyzing historical data to make informed predictions about future events. In the context of grocery demand forecasting, it offers exciting possibilities.
Utilizing predictive analytics, businesses can access a range of insights:
- Consumer Behavior Forecasts: These analytics can help businesses understand how customer preferences evolve over time, allowing them to tailor their marketing strategies.
- Stock Optimization: By predicting fluctuations in demand, grocery stores can head off stock shortages or overages, thereby reducing waste and lost sales opportunities.
- Scenario Planning: Predictive analytics can prepare businesses for various outcomes based on potential market changes, helping to mitigate risks.
In summary, the integration of AI and advancements in predictive analytics not only enhance the accuracy of forecasts but also provide a strategic advantage in the fast-moving grocery industry. Embracing these future trends means that organizations can respond more effectively to consumer needs and changes in the market, ultimately improving their operational efficiency and customer satisfaction.
Epilogue and Summary
In summing up the journey into grocery demand forecasting, it becomes clear just how pivotal this process is to not only the efficiency of supply chain management but also to customer satisfaction and profitability. Accurate forecasting empowers businesses to preemptively adjust inventory, reduce waste, and meet varying consumer needs, all of which are crucial in the competitive realm of grocery retailing.
Key Takeaways
A few core elements to keep in mind as you reflect on this analysis include:
- Data-Driven Decisions: Leveraging technology and data analytics leads to more precise predictions. The importance of relying on real-time sales data canât be understated.
- Consumer Behavior Insight: Understanding what drives customer choices, such as seasonal preferences, helps in painting a clearer picture of future demand obliquely.
- Integration of Methods: Adopting a blend of traditional and modern forecasting techniques can offer a more holistic view. Mixing qualitative insights with quantitative data tends to yield better results.
- Collaboration is Key: Departments need to work seamlessly to ensure that the insights generated from forecasting resonate across the supply chain, from procurement to sales.
- Continuous Adaptation: The landscape is ever-changing; businesses must remain flexible and responsive to both market shifts and the unpredictable nature of consumer demand.
Simply put, grocery demand forecasting is not just about numbers; itâs about understanding the pulse of the market and aligning operations to meet that rhythm.