The Jabberwacky Chatbot: An In-depth Exploration
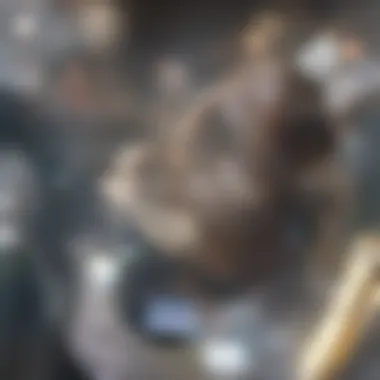
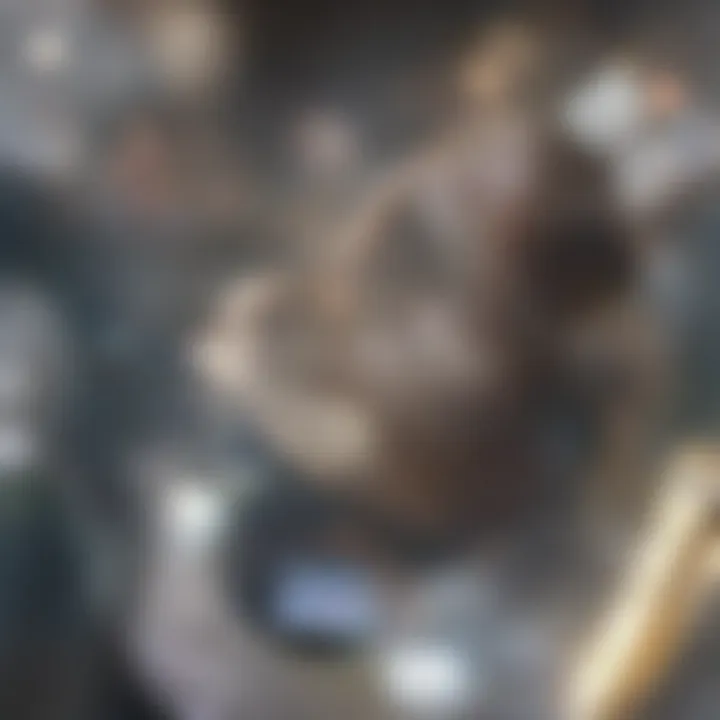
Intro
The evolution of conversational AI has seen various innovative systems emerge over the years. One of the earliest notable examples is the Jabberwacky chatbot. This chatbot operates on principles that have influenced the creation and development of many modern AI conversational agents. By understanding Jabberwacky, we can appreciate the complexities and advancements in natural language processing and artificial intelligence.
This article will provide an in-depth exploration of the Jabberwacky chatbot. It will analyze its architecture, the inherent limitations, and the nature of user interactions. Furthermore, it will connect Jabberwacky to contemporary chatbot technologies. Through these discussions, the significance of Jabberwacky in AI's history will become clear, as will its relevance in today’s technological landscape.
Key Concepts and Terminology
Definition of Key Terms
Understanding the Jabberwacky chatbot requires familiarity with specific terminology in the AI domain. Here are some essential terms:
- Conversational AI: A branch of artificial intelligence that focuses on enabling machines to communicate with humans in natural language.
- Natural Language Processing (NLP): The field of AI concerned with the interaction between computers and humans through natural language.
- Chatbot: An AI program designed to simulate conversation with human users, especially over the Internet.
Concepts Explored in the Article
In this article, we will explore several concepts related to Jabberwacky, such as its:
- Architectural design: How Jabberwacky processes and generates language.
- Limitations: The inherent challenges faced by this early AI system.
- User interactions: Engaging with users and evolving based on conversational history.
- Modern relevance: The connection to current technologies and chatbots.
Findings and Discussion
Main Findings
Through analyzing Jabberwacky, various crucial findings emerge:
- Pioneering Chatbot: Jabberwacky set a precedent in the world of conversational AI, showcasing early attempts at natural language understanding.
- User Engagement: It provided insights into the dynamics of human-computer interaction and how conversational agents adapt to user input.
- Architectural Limitations: The system demonstrated significant challenges in maintaining context and consistency in conversations.
Potential Areas for Future Research
The exploration of Jabberwacky opens several avenues for future research, such as:
- Developing more sophisticated NLP algorithms to improve contextual understanding.
- Investigating user behavior and preferences in conversational AI interactions.
- Enhancing chatbots’ learning mechanism to adapt efficiently to unique user dialogues.
The findings from Jabberwacky provide foundational insights that continue to shape AI conversational agents today.
By studying Jabberwacky, we gain valuable perspectives on the evolution of AI technologies, highlighting the ongoing relevance of early systems in shaping contemporary applications.
Foreword to Jabberwacky
Jabberwacky holds a significant place in the realm of conversational AI. Its exploration is not merely an academic exercise but a dive into the foundational elements that influenced later advancements in chatbot technology. Jabberwacky serves as a case study for understanding the progression of natural language processing and the challenges associated with creating AI that can engage in meaningful dialogue.
The importance of Jabberwacky lies in its approach to simulating human conversation. It was one of the earlier attempts to create an interactive and engaging chatbot, predating many modern AI systems. By examining Jabberwacky, one gains insights into both the innovations and limitations of early AI systems, revealing how they have shaped current understanding and practice in artificial intelligence.
Defining Chatbots
Chatbots are sophisticated software programs designed to simulate human-like conversation, enabling interaction through text or voice. Their scope ranges from simple rule-based systems to advanced AI-driven interfaces that utilize machine learning algorithms. Typically, chatbots have two primary types:
- Rule-based chatbots: These utilize predefined rules and scripted responses to answer user inquiries. Their functionality is generally limited to specific domains.
- AI-driven chatbots: These leverage complex algorithms to understand and process natural language, allowing for more nuanced and flexible interaction.
At their core, chatbots serve various purposes, including customer support, information retrieval, and even companionship. Their ability to operate continuously without human intervention brings various benefits, such as efficiency and cost-effectiveness.
Historical Context of Jabberwacky
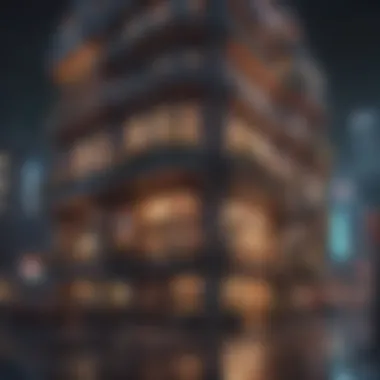
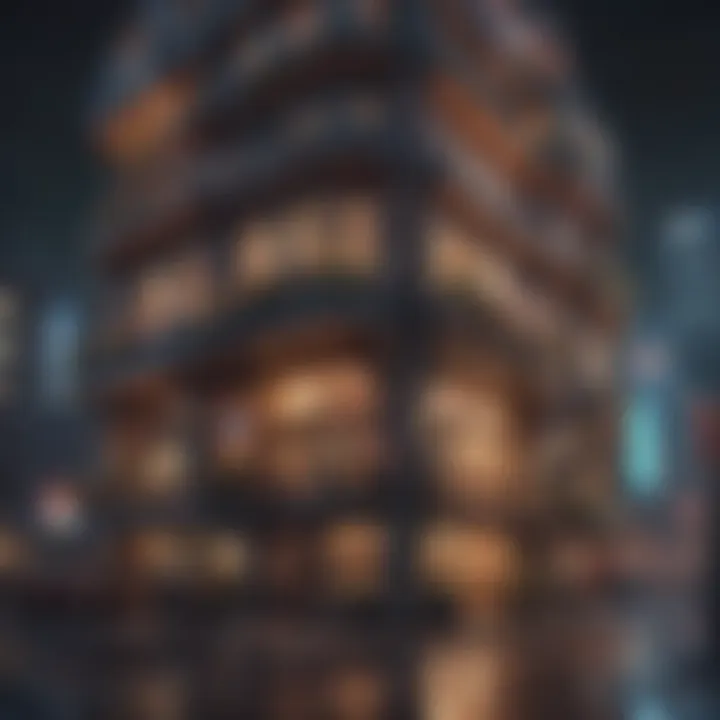
The historical context of Jabberwacky is essential to understanding its place in the evolution of conversational agents. Developed by Rollo Carpenter in the late 1980s, Jabberwacky represented a novel approach to AI that diverged from traditional methods. Unlike earlier projects, which focused primarily on symbolic reasoning, Jabberwacky emphasized learning from users through interaction.
This unique aspect allowed Jabberwacky to adapt responses based on previous conversations, developing a personality over time. It drew interest not only from tech enthusiasts but also from broader audiences who engaged with it on platforms like the internet. Jabberwacky became a prototype of how future chatbots could learn and grow, highlighting the potential for conversational AI to evolve through user engagement.
The legacy of Jabberwacky continues to influence contemporary chatbot design. Its innovative learning mechanisms paved the way for modern solutions seen in today's technologies. As AI continues to advance, the lessons learned from Jabberwacky remain relevant and instructive.
Technical Architecture of Jabberwacky
The technical architecture of Jabberwacky holds significant weight in understanding how this chatbot functions. It encompasses various algorithms and data structures that facilitate interaction, learning, and response generation. By investigating these elements, one can appreciate the complexity of Jabberwacky as an early model in the field of conversational AI. This background is crucial not only to comprehend its historical context but also to compare it effectively with modern chatbots.
Core Algorithms Used
The core algorithms of Jabberwacky reveal its operational foundations. Jabberwacky doesn’t rely on a fixed set of responses; instead, it uses a unique algorithm that allows the chatbot to generate replies based on user inputs. The primary features of these algorithms include:
- Learning Mechanism: Jabberwacky utilizes a form of memory to recall previous interactions with users. This ability enhances its conversation flow and provides more contextually relevant replies.
- Pattern Recognition: The chatbot identifies patterns in user inputs to anticipate conversational context. This recognition contributes to smoother interactions and a more engaging user experience.
- Response Generation: Utilizing a probabilistic model, Jabberwacky generates responses based on historical dialogues. This means that the chatbot can produce diverse replies, making conversations feel more natural and dynamic.
These algorithms combined create an adaptable system that strives to mimic human-like conversation.
Data Structures and Storage
The data structures employed in Jabberwacky are critical for managing conversational data and ensuring smooth interactions. Key aspects of how Jabberwacky handles data include:
- Storage Mechanisms: Jabberwacky organizes its information in a way that allows efficient retrieval of past conversations. The use of trees, arrays, and hash tables aids in structuring these interactions.
- Input Handling: The chatbot captures user inputs and stores them for future reference. This facilitates learning and allows it to reference earlier exchanges, thereby enhancing continuity in conversation.
- Response Database: Jabberwacky maintains a sizable database of responses. This database not only supports quick reply generation but also serves as a foundation for learning new conversational patterns over time.
The architecture behind Jabberwacky supports a system that can learn from its users, adapt to different conversational styles, and ultimately engage more effectively. Understanding its technical framework provides insight into the significant role Jabberwacky played in paving the way for contemporary chatbots.
"Jabberwacky reminds us of the challenges and achievements in the domain of conversational AI, illustrating a path toward more sophisticated systems that exist today."
User Interactions with Jabberwacky
User interactions with Jabberwacky represent a crucial aspect of understanding how conversational AI can emulate human-like communication. Jabberwacky is designed to engage users through conversation, making it essential to analyze how it interprets and responds to input from users. The effectiveness of these interactions often determines users’ perceptions of the chatbot’s capabilities and limitations.
Effective user interaction is rooted in several key elements. First, the ability to maintain context in a conversation is vital. Jabberwacky does this by attempting to reference previous statements made during the same session. This creates a semblance of continuity that mimics natural human dialogue. Additionally, Jabberwacky utilizes a vast database of prior conversations, allowing it to draw upon diverse responses based on user input.
Another important aspect is the diversity of engagement. Jabberwacky engages users from various backgrounds, which presents both opportunities and challenges. Users with different expectations may find the interactions enjoyable or frustrating, impacting overall satisfaction. Understanding these dimensions helps developers refine user experiences going forward.
Moreover, collecting and analyzing user feedback allows Jabberwacky to adapt. In practice, this other data can guide future interactions, enhancing the chatbot’s ability and relevance within changing conversational contexts. Each interaction not only serves as immediate entertainment but also feeds back into the system to improve performance.
Understanding Conversation Flow
Conversation flow within Jabberwacky is instrumental in how effectively it mimics human conversation. The flow consists of the logical sequence of exchanges, where it must interpret both the context and intent behind user queries. Jabberwacky’s ability to handle various conversational paths directly affects user retention and engagement.
At the core, conversation flow can be impacted by multiple factors, such as user prompts. The structure of user inputs can either prompt direct responses or lead to more complex follow-up questions. By analyzing these prompts, Jabberwacky aspires to respond appropriately—a process that involves evaluating syntax and semantics to discern intent.
Furthermore, maintaining engagement requires managing transitions between topics. A seamless shift enhances the conversational experience. In contrast, abrupt or disjointed responses may deter further interaction. Overall, effective conversation flow hinges on a balance between context retention and adaptive response generation.
Patterns in User Engagement
Patterns in user engagement reveal insights into preferences, behaviors, and expectations in interactive settings. By examining these patterns, developers can understand how users interact over time, informing modifications to improve engagement with Jabberwacky.
The most common forms of engagement include:
- Duration of sessions: Users often favor longer conversations if more relevant content is provided.
- Response length: There is a tendency for users to expect concise replies that are straightforward and relevant to the preceding exchanges.
- Revisit rate: A high revisit rate often indicates user satisfaction. This metric helps gauge the chatbot's effectiveness in maintaining user interest.
Additionally, engagement can fluctuate based on the complexity of topics introduced or the emotional tone of user inputs. Positive interactions may elicit extended conversations, while confusion or frustration tends to result in abrupt ends.
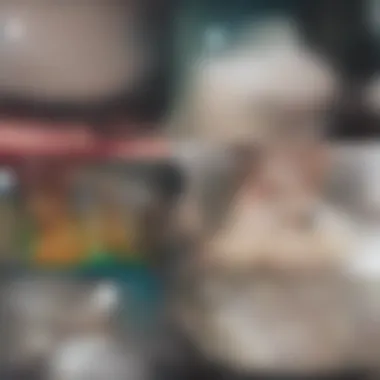
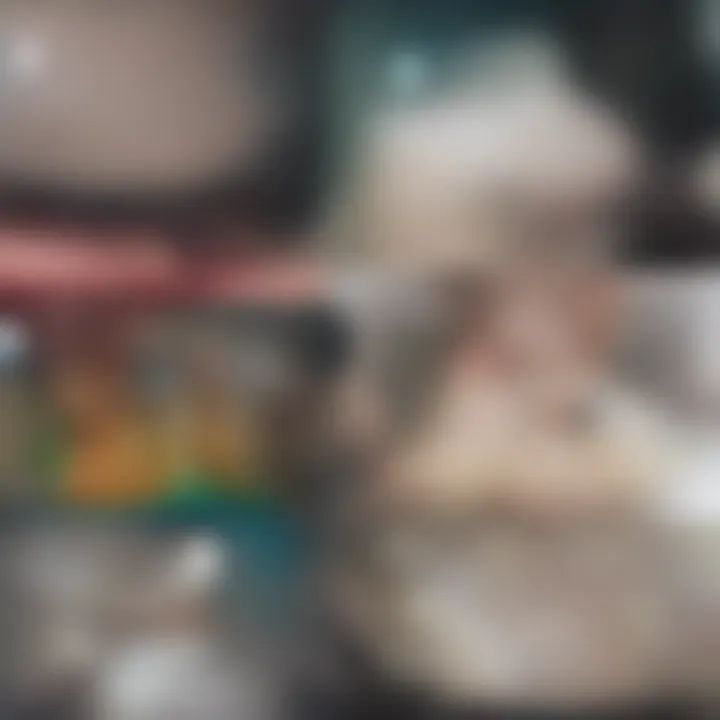
Limitations of Jabberwacky
Understanding the limitations of Jabberwacky is vital, as it highlights challenges that early chatbots face in the field of conversational AI. Despite its pioneering role in the evolution of chatbots, Jabberwacky reveals several shortcomings, especially in terms of natural language processing and user interaction. Acknowledging these limitations provides essential lessons for current and future developments in AI systems.
Challenges in Natural Language Processing
Jabberwacky demonstrates significant challenges in natural language processing, which eventually impacts its effectiveness in conversation. First, its reliance on pattern recognition means that it struggles to comprehend context and nuance in user inputs. This can lead to misinterpretation of user intent. Moreover, Jabberwacky employs a keyword-based system which is simplistic, limiting its ability to understand more complex sentences or the subtleties of human conversation.
Additionally, Jabberwacky often generates responses that feel mechanical and formulaic. This can detract from the user experience as users might find interactions unengaging. Without the capacity for deep understanding, Jabberwacky cannot manage ambiguity in conversation. Often, it leads to frustrating exchanges, where users do not feel heard or understood. The problem is compounded by a limited vocabulary, which constrains the breadth of conversations it can facilitate.
"A chatbot that lacks deep comprehension can often mislead and confuse users, negating the advantages of AI."
These challenges suggest that while Jabberwacky opened paths for future chatbot development, it was bound by its limitations. This adds to the discussion in modern AI whereby enhancing conversational capabilities is crucial.
User Experience Constraints
User experience with Jabberwacky also reveals various constraints that prevent effective dialogue. One notable aspect is the lack of personalization. Users may find conversations monotonous or impersonal since the chatbot cannot adapt to individual preferences or prior interactions. This absence of continuity can lead to a disjointed experience, where each exchange feels isolated rather than part of an ongoing dialogue.
Another important consideration is the interface design. While it was innovative for its time, the lack of multi-modal interaction limits how users can engage with Jabberwacky. Modern chatbots often incorporate various media forms, such as voice, text, and visuals, enriching the interaction. In contrast, Jabberwacky offers a more basic, less immersive experience.
Furthermore, response time plays a crucial role in user satisfaction. Delays in generating responses can cause frustration and disconnect, leading users to abandon conversations prematurely. As a result, Jabberwacky illustrates the significant gap between user expectations and reality during interactions, emphasizing the importance of optimizing user experience in chatbot design.
In summary, the limitations faced by Jabberwacky in natural language processing and user experience underscore critical lessons in the evolution of conversational AI. Acknowledging these flaws not only informs present advancements but also shapes future innovations.
Comparative Analysis with Modern Chatbots
The role of comparative analysis with modern chatbots is critical in understanding the evolution of conversational AI. This section emphasizes how an examination of Jabberwacky vis-a-vis contemporary chatbots can provide insights into advancements and challenges faced by this technology over time. Analyzing various chatbots focuses on examining significant technological shifts and understanding user expectations in the digital age.
Advancements in Conversational AI
The progress of conversational AI from Jabberwacky to current sophisticated models is remarkable. Jabberwacky utilized a simple response mechanism based on user input, leveraging a basic form of pattern matching and association. Modern chatbots, however, employ complex algorithms and neural networks to achieve an advanced level of understanding. Notably, models like OpenAI's ChatGPT or Google's LaMDA exhibit deep learning capabilities that allow for more nuanced conversation.
Some significant advancements include:
- Natural Language Understanding (NLU): Advanced models now better comprehend context, intent, and sentiment.
- Data-driven Learning: Modern systems learn from large datasets, enabling them to adapt continuously and improve user interactions.
- Contextual Memory: Unlike Jabberwacky, which had limited contextual awareness, current chatbots can remember past interactions for a cohesive conversation.
These advancements indicate a shift from basic programmed responses to intelligent, dynamic interaction that improves the user experience significantly.
Use Cases in Different Sectors
The application of chatbots in diverse sectors underlines their versatility and importance in today's technology landscape. While Jabberwacky was primarily a novelty offering limited practical applications, modern chatbots serve in various professional fields. Here are some prominent use cases:
- Healthcare: AI chatbots assist in diagnosis, appointment scheduling, and patient follow-ups. Tools like Babylon Health and Zocdoc represent this advancement well.
- Customer Service: Bots like Intercom and Zendesk enhance customer interaction by providing instant support, FAQs, and troubleshooting without human intervention.
- Education: Platforms such as Duolingo utilize chatbots for language learning by simulating conversation, making learning interactive.
- Finance: Chatbots like Cleo offer financial advice and budgeting assistance, showcasing their role in personal finance management.
These applications demonstrate the transformative impact modern chatbots have across industries, vastly outpacing the early capabilities of Jabberwacky. It shows how technology has refined the user experience, addressing specific needs and preferences
Modern chatbots are not just conversational agents; they are integral tools for enhancing efficiency and engagement across various sectors.
The Significance of Jabberwacky in AI History
The Jabberwacky chatbot is more than just a curious conversational program; it is a beacon in the evolving narrative of artificial intelligence. This section will explore its significance through two lenses: cultural impact and the legacy it left for future AI systems. By understanding these elements, one can appreciate how Jabberwacky has influenced the landscape of AI as we know it today.
Cultural Impact and Recognition
Jabberwacky emerged during a period when chatbots were an experimental curiosity, capturing both public attention and academic interest. Its engaging approach to conversation provided users a glimpse into the potential of artificial intelligence in social interactions. Unlike many of its predecessors, which relied on strict algorithms and could be easily predicted, Jabberwacky employed a more fluid and less structured method. This made conversations feel lively and unpredictable.
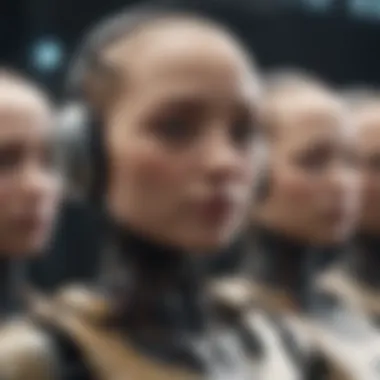
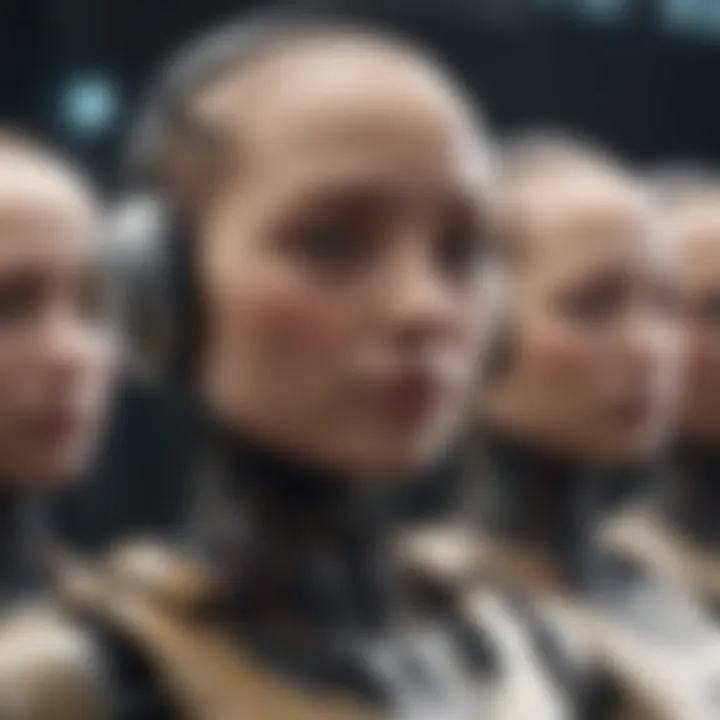
The recognition of Jabberwacky can be observed across multiple domains:
- Media Exposure: It received coverage in various media outlets, stirring conversations about what it means for a computer to simulate human-like interaction.
- Competitions: In 2005, it won the Loebner Prize, a notable accolade in the realm of artificial intelligence. This win prompted discussions on the future capabilities of chatbots.
- Public Engagement: Users were drawn to Jabberwacky not just for its novelty, but for its capacity to articulate complex exchanges, thus creating a form of digital companionship.
"The truth about AI is that it represents a milestone rather than an endpoint."
Legacy and Influence on Future AI Systems
Jabberwacky's contributions do not end with its cultural impact. Instead, it has laid vital groundwork for future artificial intelligence innovations. Several key aspects mark its legacy:
- Technological Foundation: Jabberwacky’s design and architecture have informed the development of modern chatbots. Its approach highlights the importance of learning and adapting through user interactions.
- Natural Language Processing Advancement: The challenges it faced are echoed in contemporary discussions about natural language understanding. Researchers continually reference Jabberwacky to analyze the persistent issues within AI communication.
- Inspiration for New Models: Later systems, such as OpenAI's ChatGPT, have drawn on the inspiration fostered by early models like Jabberwacky to refine their conversational abilities.
Future Developments and Trends in Chatbots
The future of chatbots is both promising and complex. As we explore further, it becomes clear that Jabberwacky lays some groundwork for understanding these developments. The evolution of these systems will not just depend on technical advancements but also on their societal implications. The increasing demand for smarter and more interactive bots is driving developments in both the underlying technology and their integration into daily life.
Emerging Technologies in AI
Recent trends highlight a shift toward more sophisticated artificial intelligence. Technologies such as machine learning, natural language processing, and deep learning are reshaping chatbot functionality. For instance, advancements in transformer models, such as those used in Google’s BERT or OpenAI's ChatGPT, allow chatbots to understand and generate human-like text more effectively.
Additionally, multi-modal AI is emerging. This combines text, audio, and visual inputs, allowing chatbots to engage in more nuanced conversations.
- Key Technologies Shaping the Future:
- Machine Learning Algorithms: Continuously learn from user interactions.
- Natural Language Processing: Improve understanding of nuanced language.
- Deep Learning Frameworks: Enables complex pattern recognition in discussions.
- Voice Recognition: Enhancing interaction through spoken language.
Collaboration between companies and research institutions is also vital in this context. With personal assistants like Amazon Alexa and Google Assistant setting high expectations, the industry is being compelled to enhance user experience. By focusing on seamless integration, businesses will look towards deploying chatbots that can handle diverse queries with precision. Such trends not only improve the chatbot's relevance but also their practical applicability across different sectors.
Ethics and Responsible AI Implementation
As chatbots become more prevalent, ethical considerations will significantly shape their future. An environment must be established where chatbots operate not just efficiently but also responsibly. Ethical frameworks can guide the development of these systems to ensure they align with societal values and legal standards.
Aspects to consider include:
- Data Privacy: How user data is collected, stored, and used. This is critical to maintain trust.
- Transparency: Users should know when they interact with a chatbot and understand its capabilities.
- Bias Mitigation: Ensuring that AI systems do not perpetuate existing biases present in training data, fostering fairness in interactions.
It is crucial for developers to engage with ethicists and social scientists in the development process. This collaboration can facilitate comprehensive guidelines, ensuring that future chatbot technologies do not compromise ethical standards.
"The implementation of ethical AI is not just a good practice; it is essential for the sustainable advancement of technology in society."
In summary, the landscape of chatbots will be shaped by emerging technological advancements and increasing ethical scrutiny. As we anticipate the future, these elements incorporate an intricate interplay of innovation and responsibility, leading to more capable and conscientious conversational agents.
The End: Reflecting on the Journey of Jabberwacky
The exploration of Jabberwacky culminates in a thorough understanding of its role in conversational AI. Jabberwacky is not merely a historical footnote; it embodies the evolution of human-computer interaction. Its journey sheds light on the early challenges and triumphs faced by developers in the field of natural language processing.
Recapping Key Insights
Reflecting on the discussions throughout this article, several key insights surface:
- Innovative Architecture: Jabberwacky employed an adaptive learning model that allowed it to improve responses based on user interaction. This laid groundwork for future AI applications.
- Cultural Significance: The chatbot's development was a key moment in the AI narrative. It inspired later projects and drew attention to the potential of conversational agents.
- Limitations Highlighted: While Jabberwacky made strides, it also exposed limitations in technology, such as context understanding and user engagement. These weaknesses continue to inform ongoing research in conversational AI.
Moreover, the analysis of user interactions showcased the importance of maintaining human-like dialogue. This characteristic continues to be an essential factor for success in modern chatbots.
Looking Ahead: The Role of Chatbots in Society
As we consider the future, chatbots like Jabberwacky pave the way for increasingly nuanced AI systems. Their implications are broad:
- Integration in Daily Life: Chatbots are finding roles in customer service, healthcare, and even education. The ability to handle inquiries efficiently speaks to their growing importance.
- Ethics and AI: The evolution from Jabberwacky prompts critical discussions on ethical implementations. As AI becomes embedded in daily life, understanding moral frameworks and user privacy will be paramount.
- Continued Innovation: Emerging technologies promise to improve the capabilities of chatbots. Developments in machine learning and natural language processing will shape the next generation of conversational AI.
In summary, the journey of Jabberwacky provides valuable lessons, not only on technological advancement but also on societal impacts. The landscape of AI continues to shift, and understanding past innovation is crucial to navigating future challenges.