Measuring Clinical Outcomes in Healthcare Effectively
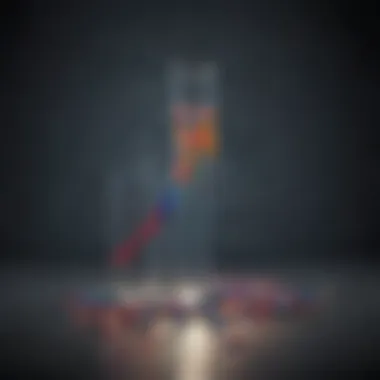
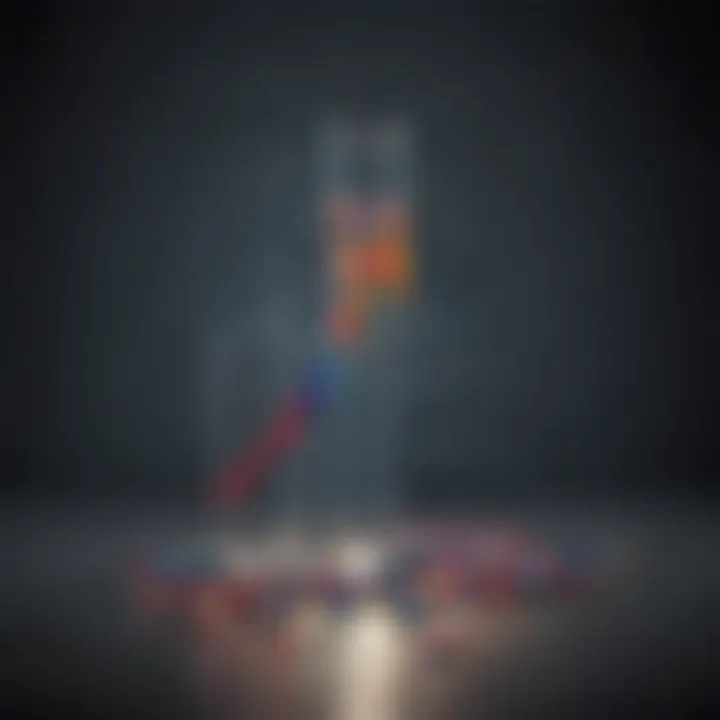
Intro
In the continuously evolving landscape of healthcare, understanding clinical outcomes has become a focal point for medical professionals, researchers, and policy makers alike. The measurement of clinical outcomes isn't just some academic exercise. Rather, it's a crucial element that determines how effective a treatment or intervention is in the real world. Consider it the difference between just treating the symptom versus truly addressing the underlying issue. With rising healthcare costs and increasing scrutiny over treatment efficacy, the need for quantifiable results has never been more pressing.
Measuring clinical outcomes evaluates the effectiveness of various therapies, informs best practices, and ultimately impacts patient care quality.
Key Concepts and Terminology
Definition of Key Terms
Understanding the terminology is integral in grasping the complex nature of clinical outcomes. Here are some pivotal terms to kick off this discussion:
- Clinical Outcomes: These refer to the end results of health care practices and interventions, which typically encompass metrics like symptom relief, recovery rates, and quality of life.
- Data Collection: A systematic approach for gathering information used to assess and evaluate clinical outcomes. This can be achieved through methods ranging from surveys to electronic health records.
- Effectiveness: It denotes how well a specific intervention works in actual practice, as opposed to controlled settings in clinical trials.
Concepts Explored in the Article
This article doesn't shy away from dissecting various methodologies used in measuring clinical outcomes. Hereās a brief look at some of the key areas we will explore:
- Various metrics to gauge treatment success and how they align with patient perceptions.
- The challenges associated with data collection in diverse clinical settings.
- The implications of outcome measurement on patient care and healthcare policies.
Findings and Discussion
Main Findings
Throughout our examination of clinical outcomes, several key findings emerge:
- Significance of Patient Perspective: Traditional metrics often overlook patient-reported outcomes, which can be equally, if not more, crucial for understanding treatment effectiveness.
- Data Collection Challenges: There's a vast difference between theoretical data collection protocols and what happens in practice. Issues such as incomplete data, variability in reporting, and the timeliness of data can skew results.
- Implications for Policy: Outcome measures don't just inform clinical decisions, they have far-reaching implications for healthcare policies, funding priorities, and the direction of medical research.
Potential Areas for Future Research
As clinical outcomes measurement evolves, there are several areas ripe for further exploration:
- Integration of Technology: The role of Artificial Intelligence and machine learning in predicting clinical outcomes and improving data accuracy.
- Cross-Domain Comparisons: Investigating outcomes across different healthcare systems and practices can offer insights on best practices.
"The challenge in research is not only to find answers but to ask the right questions first."
Lastly, as the landscape continues to shift, staying abreast of emerging methodologies and integrating new technologies will play a pivotal role in enhancing our understanding of clinical outcomes. The work does not end here; rather, it offers a launchpad for ongoing inquiry and refinement in measuring what truly matters in healthcare.
Prelims to Clinical Outcomes
In the realm of healthcare, understanding clinical outcomes is paramount. The effectiveness of any treatment or intervention can only be gauged through the lens of its outcomes. What would be the point of medical advancement if we did not measure the real-world impacts of treatments on patients? This section shines a spotlight on the fundamental premise of measuring outcomes.
Defining Clinical Outcomes
To put it simply, clinical outcomes refer to the tangible effects of healthcare interventions on patients' health status. These outcomes can manifest in various ways, encompassing everything from physiological changes to subjective improvements in a patient's quality of life. For instance, a patient recovering from knee surgery might experience decreased pain and improved mobility ā these are clear clinical outcomes that signal the procedure's success.
Defining clinical outcomes goes beyond just the basic effects; it requires an understanding of context. Outcomes must be relevant and measurable, tied directly to the goals of treatment protocols. Outcomes, therefore, can be classified into several categories:
- Clinical Outcomes: Objective measures like mortality rates or recovery times.
- Patient-Reported Outcomes (PROs): These are subjective reports from patients, providing insights into their health status and quality of life post-intervention.
- Functional Outcomes: Measures of a patient's ability to perform daily activities, which often reflect the general state of health.
When these outcomes are well-defined, they lay the groundwork for better assessments in clinical practice, allowing healthcare providers to refine treatment plans continually.
Significance of Measuring Outcomes
Measuring clinical outcomes holds significant weight not just for practitioners but for the patients themselves as well. Ultimately, all efforts in healthcare gear towards enhancing patient wellbeing. By quantifying outcomes, healthcare professionals can make informed decisions that foster improvement. Among the key benefits of measuring outcomes are:
- Evidence-based Practices: Data collected from outcome measurements serve as the bedrock for developing evidence-based guidelines, which streamline the decision-making process.
- Quality Improvement: Continuous measurement and evaluation bring forth insights that identify areas of improvement, fostering a culture of perpetual progress within healthcare settings.
- Patient Satisfaction: When patients experience tangible benefits from their treatment, their satisfaction levels rise. Understanding and addressing each patient's needs can ultimately enhance their experience.
Measuring outcomes can also serve as a bridge for communication between healthcare providers and patients. It allows both parties to track progress and discuss any necessary adjustments in treatment. By honing in on these metrics, practitioners can reinforce a sense of partnership in the care process.
"Healthcare is not just about curing illnesses; itās about improving lives."
The Evolution of Outcome Measurement
Measuring clinical outcomes is not just a static affair; it's a journey that has transformed over time. Understanding the evolution of outcome measurement is crucial to grasp how far we've come and where we're headed. This section highlights the historical context and current trends, illuminating the benefits and considerations involved.
Historical Perspectives
The roots of outcome measurement trace back to the early 20th century when clinical practices were rudimentary at best. Back then, treatment evaluations relied heavily on anecdotal evidence and observational studies. Practitioners had a limited understanding of what constituted a successful treatment outcome. The focus was often on survival, with little consideration of the quality of life experienced by patients.
As the decades rolled on, the medical field began to adopt more systematic ways of documenting outcomes. The introduction of standardized medical terminology and classification systems, like the International Classification of Diseases (ICD), marked a significant milestone. This standardization allowed for clearer communication, better data collection, and ultimately improved patient care.
Fast forward to the late 20th century, a time when evidence-based medicine gained traction. Randomized controlled trials (RCTs) emerged as the gold standard for evaluating clinical interventions. This development was revolutionary; it emphasized not just the efficacy of treatments but also the importance of objectively measuring patient outcomes. In this environment, statistical methods became indispensable tools for researchers and healthcare professionals alike, laying the groundwork for today's sophisticated measurement techniques.
Thus, it is evident that the historical perspectives on outcome measurement reveal a constant striving for precision and clarity. From anecdotal to evidence-based practices, the path has been paved with innovations aimed at enhancing patient care.
Current Trends in Measurement Techniques
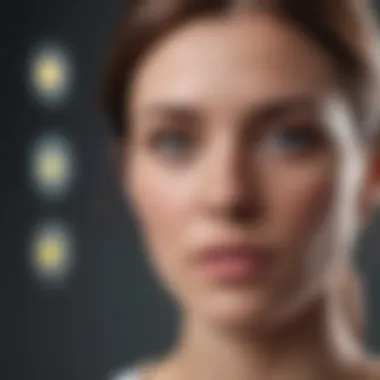
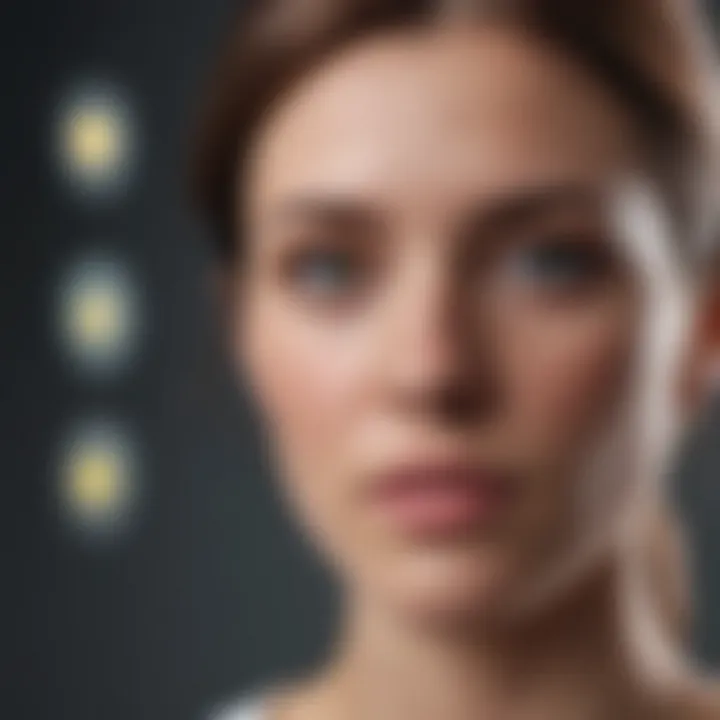
In our modern era, current trends in measurement techniques are shaped by technology and a growing emphasis on patient-centered care. Healthcare professionals are increasingly adopting both quantitative and qualitative methods to gather comprehensive data on clinical outcomes.
The emergence of electronic health records (EHRs) has simplified data collection and facilitated the tracking of patient outcomes over time. With this technology, healthcare providers can easily access vast amounts of data. Moreover, patient-reported outcomes (PROs) have gained popularity, offering insights directly from patients about their health status and satisfaction with treatments.
In terms of measurement methodologies, weāre seeing a blend of traditional methods with innovative approaches. For example, mobile health applications have been leveraged to collect real-time data from patients, providing a more dynamic understanding of treatment effects. Tools like machine learning and artificial intelligence are also making waves, allowing healthcare analytics to predict patient outcomes with greater accuracy by analyzing complex datasets.
While these advancements are promising, they also present challenges. Issues like data privacy, the accuracy of self-reported information, and the need for standard validation methods are ongoing concerns that professionals must address.
"In the healthcare landscape, the evolution of outcome measurement serves as a testament to our commitment to bettering patient care through informed decision-making."
Methodologies for Measuring Clinical Outcomes
Understanding methodologies for measuring clinical outcomes is crucial. It offers a structured guide to the numerous strategies and techniques available to assess the effectiveness of medical interventions. With the right methodologies, health care professionals can rely on data-driven insights that contribute to better patient outcomes and enhanced treatment protocols. Moreover, selecting an appropriate approach often leads to a more accurate understanding of a patient's health status and treatment response.
Quantitative Approaches
Utilization of Statistical Methods
Utilization of statistical methods plays a pivotal role in quantitative research within clinical outcomes. This technique allows researchers and clinicians to analyze numerical data and derive meaningful conclusions. By employing various statistical tools, healthcare professionals can identify trends, correlations, and variations significant to patient health.
A key characteristic of statistical methods is its systematic approach. This method utilizes large datasets to draw conclusions about the effects of specific treatments or interventions. The ability to quantify clinical outcomes means that healthcare decisions can be more confidently made based on substantial numbers rather than subjective interpretation.
However, these methods do come with unique challenges. While statistical methods are beneficial for their objective nature, they can also mask the nuances of individual patient experiences, possibly leading to over-generalizations. Therefore, it's of utmost importance to balance the use of statistical analysis with qualitative insights to achieve comprehensive patient understanding.
Longitudinal vs. Cross-sectional Studies
When measuring clinical outcomes, understanding the differences between longitudinal and cross-sectional studies is vital. Longitudinal studies follow the same subjects over a period, enabling researchers to observe changes and development in clinical outcomes over time. In contrast, cross-sectional studies provide a snapshot by examining a population at a single point in time.
The main benefit of longitudinal studies lies in their ability to track progression and establish causation, which is invaluable for identifying the long-term impacts of treatments. This method is particularly useful in chronic disease management, where understanding changes over time can inform ongoing treatment plans. However, they can be resource-intensive and time-consuming to conduct.
Cross-sectional studies, on the other hand, are often simpler and quicker to administer. They can provide immediate insights about a populationās health outcomes.
However, this method lacks the depth of longitudinal studies. Results might not demonstrate causative relationships due to the simultaneous data collection of variables. Each approach has strengths and weaknesses, necessitating careful consideration based on the specific research question at hand.
Qualitative Approaches
Patient-Reported Outcomes
Patient-reported outcomes (PROs) capture the patients' perspectives on their health condition. By using PROs, clinicians can gain insights directly from those affected, thus reflecting the real-world impact of treatment from the patientās angle. This is especially important in areas like chronic pain management where subjective experience vastly influences perceived treatment efficacy.
The key advantage of including PROs in clinical outcome measurement is that they put the patient at the center. This approach helps health professionals understand how patients perceive their condition and the effectiveness of their treatment. However, some may argue that PROs can be influenced by factors like mood or contextual elements, creating variability that complicates results interpretation.
Interviews and Focus Groups
Interviews and focus groups involve direct engagement with patients to discuss their experiences and perceptions regarding their health outcomes. These qualitative methods offer rich, detailed insights that quantitative approaches may overlook. The ability to ask open-ended questions allows for flexibility in responses, leading to an in-depth understanding of patient perspectives.
One significant characteristic of these approaches is their emphasis on narrative. Patients have the opportunity to tell their stories, providing context that numbers alone cannot capture. On the downside, analyzing qualitative data can be labor-intensive and subjective, potentially leading to biases in interpretations depending on the reviewer.
In sum, both quantitative and qualitative methods serve unique and essential roles in measuring clinical outcomes. Choosing the right approach requires careful thought about the study goals and the characteristics of the patient population involved, ultimately aiming for a comprehensive understanding of clinical effectiveness.
Data Collection Techniques
Data collection techniques are crucial in clinical outcomes measurement as they provide the foundation for understanding the efficacy of healthcare interventions. The methods utilized in gathering data can significantly influence the quality and reliability of the results obtained. With myriad approaches available, the careful selection and execution of these techniques can ensure that the data reflects the actual patient experiences and treatment effects.
When it comes to measuring clinical outcomes, it's often a balancing act. You want to capture meaningful information without overwhelming participants or compromising data integrity. Employing rigorous data collection methods serves not only to reinforce the credibility of the findings but also to directly impact patient care improvements.
Surveys and Questionnaires
Surveys and questionnaires are among the most frequently used tools in clinical outcomes measurement. They offer a systematic way to gather data directly from patients regarding their experiences, satisfaction, and health status. One of the undeniable benefits of using surveys is their ability to reach a large number of participants quickly, often at a low cost. This scalability can be especially beneficial when assessing outcomes across diverse populations.
When designing surveys, researchers must be meticulous in crafting questions. Clarity is key; ambiguous language can yield unreliable data. Furthermore, the format of the questionnaire can influence responses. Multiple choice, Likert scales, and open-ended questions each present distinct advantages and might elicit different kinds of information.
- Pros of using surveys:
- Cons of using surveys:
- Efficient data collection from many respondents
- Flexibility in question types
- Can be distributed in various formats (online, paper, etc.)
- Potential for response bias
- Limited depth of information compared to qualitative methods
In essence, surveys and questionnaires act as a bridge, connecting healthcare providers to patients' realities and experiences.
Clinical Trials and Registries
Clinical trials and registries play a pivotal role in the landscape of clinical outcomes measurement. They serve to capture data related to specific interventions and populations over time, enabling an in-depth understanding of treatment effects. Clinical trials, typically prospective studies, allow researchers to evaluate the efficacy and safety of new treatments by following a defined group of patients through various stages.
Registries, on the other hand, collect data on patients with specific conditions or undergoing certain treatment protocols, creating a repository of information that can be invaluable for understanding long-term outcomes. Such data can illuminate trends and help establish best practices.
- Advantages of clinical trials:
- Shortcomings of clinical trials:
- Benefits of registries:
- Challenges of registries:
- Rigorous scientific methodology
- Opportunities for direct patient interaction and monitoring
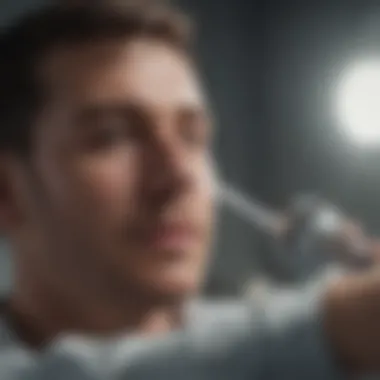
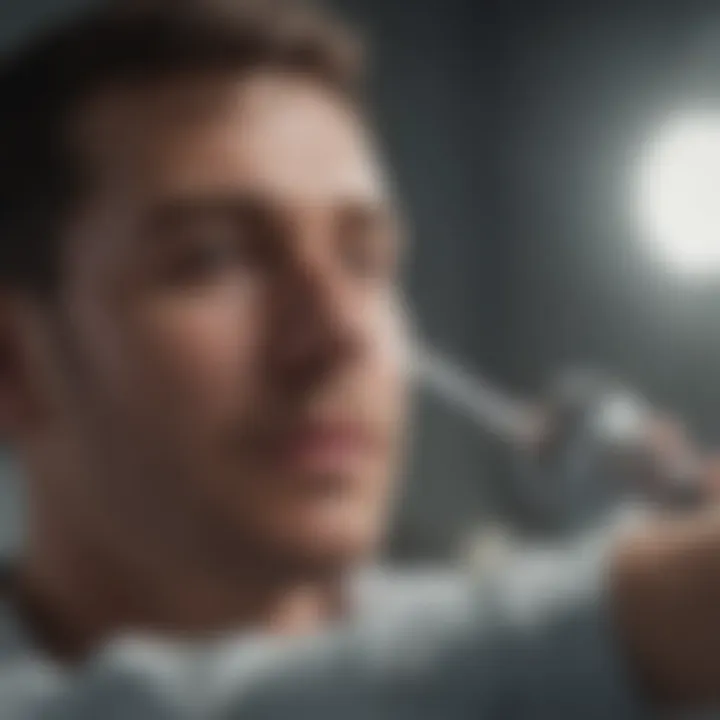
- Often time-consuming and costly
- Strict eligibility criteria can limit generalizability
- Broad representation of diverse patient populations
- Ability to observe long-term outcomes in real-world settings
- Data quality varies across registries
- Potential for incomplete data collection
Key Indicators of Clinical Outcomes
Measuring clinical outcomes is not just a routine exercise; itās the backbone of effective healthcare. The key indicators of clinical outcomes provide invaluable insights into treatment effectiveness and areas where improvement is essential. These indicators serve various purposes from informing healthcare policies to guiding individual patient care strategies. By focusing on pertinent measures, healthcare providers can fine-tune their interventions to get the best bang for their buck.
When it comes to identifying key indicators, two stand-outs that often take center stage are mortality rates and quality of life measures. These metrics can act as a window, allowing us to peek not just into the efficacy of treatments but also into the broader implications of healthcare practices.
Mortality Rates
Mortality rates are perhaps one of the most straightforward indicators of clinical outcomes. This measure reflects the number of deaths in a specified population during a particular time period. Understanding these rates can be critical for gauging the effectiveness of medical interventions, especially in severe conditions like cancer or heart disease. Notably, a lower mortality rate often signifies effective treatment protocols and can enhance confidence in healthcare systems among patients.
However, thereās more to this figure than meets the eye. Mortality rates can be influenced by various factors including:
- Demographics: Age, gender, and socio-economic status affect general health and mortality.
- Co-morbidities: The presence of multiple health issues can complicate treatment outcomes.
- Access to Care: Areas with limited healthcare access may report higher mortality rates.
Consequently, when interpreting mortality rates, it is paramount to consider these contextual elements. A statistic can tell a story, but it's the nuances behind it that provide a full picture.
Quality of Life Measures
On the flip side, we have quality of life measures, which put patient experiences at the forefront. Unlike mortality rates that focus solely on survival, these measures gauge the impact of disease and treatment from the patientās perspective. Factors such as physical functioning, mental health status, and social well-being are included in these assessments.
Quality of life indicators are cornerstones in chronic disease management. For instance, a cancer patient may be surviving longer due to successful treatment, but if the treatment leads to debilitating side effects or significantly reduces their quality of life, the outcome may leave much to be desired. This echoes the sentiment that merely extending life is not the only goal; the quality of that life is equally vital.
Evaluating quality of life can be done through various validated tools such as surveys and questionnaires, and it typically examines aspects like:
- Physical Health: Energy levels, pain, and ability to perform daily activities.
- Emotional Well-being: Feelings of distress, anxiety, or depression related to health.
- Social Functioning: Effects of illness on relationships and social activities.
"Measuring clinical outcomes through indicators like mortality and quality of life allows healthcare providers to tailor treatments that resonate with the patientās real-world experience."
In summary, the interplay between mortality rates and quality of life measures creates a rich tapestry of insights about clinical outcomes. Understanding both these indicatorsārather than focusing solely on one or the otherāfosters a more holistic approach to evaluating and improving patient care. As the healthcare landscape evolves, the emphasis on these key indicators will only intensify, driving efforts towards more meaningful patient experiences.
Analyzing and Interpreting Clinical Data
In understanding clinical outcomes, the process of analyzing and interpreting clinical data cannot be overlooked. It serves as a foundational stone for drawing meaningful insights from various studies and trials. The crux of this practice lies in the ability to transform raw data into useful information that informs healthcare decisions and improves patient care. This section explores two critical components of this process: statistical analysis techniques and the challenges that arise during data interpretation.
Statistical Analysis Techniques
Statistical analysis techniques are essential tools in the hands of researchers and analysts. These methods help to quantify clinical outcomes and identify trends that might otherwise go unnoticed. Here are some key statistical techniques commonly applied in clinical research:
- Descriptive Statistics: These provide a summary of the data. This includes measures of central tendency like mean and median, as well as measures of variability such as standard deviation. Descriptive statistics set the stage for understanding the basic characteristics of the dataset.
- Inferential Statistics: This statistical branch helps to draw conclusions that extend beyond the immediate data. Techniques like t-tests, chi-square tests, and ANOVA are employed to determine if observed effects are statistically significant.
- Regression Analysis: This method assesses the relationship between variables. It helps in predicting outcomes based on one or multiple predictors, giving deeper insights into how various factors influence clinical results.
- Survival Analysis: Particularly relevant in clinical studies, survival analysis evaluates the time until an event occurs, such as patient survival or disease recurrence. Techniques like Kaplan-Meier curves are commonly used in this context.
The choice of statistical analysis technique often depends on the research question and the nature of the data. Utilizing the right methods ensures that the findings are not just numbers, but rather meaningful interpretations that can drive decisions in clinical settings.
Data Interpretation Challenges
Data interpretation is a fine art, often fraught with challenges. Even the most carefully collected data can lead to misleading conclusions if not interpreted properly. Some key challenges include:
- Bias: Bias can seep into data collection and analysis stages. For instance, selection bias can occur if the sampled population does not accurately represent the broader group, skewing results.
- Confounding Variables: These are extraneous variables that can muddy the waters of analysis. Ignoring these can lead to erroneous assumptions about causality, masking true relationships between treatment and outcomes.
- Data Quality: The quality of data collected impacts interpretation drastically. Missing data, inaccurately recorded information, or errors in data entry can distort findings, leading to flawed conclusions.
- Complexity of Medical Conditions: Many clinical outcomes are influenced by a multitude of factors. Simplifying complex conditions into single metrics can overlook significant nuances that could inform better patient care.
"Data doesnāt speak for itself; it tells a story that must be interpreted through careful analysis."
For researchers, overcoming these challenges requires a robust understanding of both the data and the broader clinical context. Engaging with these difficulties head-on can lead to far more reliable conclusions, ultimately improving patient outcomes and guiding future research endeavors.
In summary, the realm of analyzing and interpreting clinical data is intricate and essential to advancing healthcare. Employing diverse statistical techniques while being cognizant of interpretation challenges allows for a richer exploration of clinical outcomes. This not only enhances the validity of research findings but also lays the groundwork for informed clinical practice.
Impact of Outcome Measurement on Patient Care
Outcome measurement has a profound ripple effect in the healthcare sector, fundamentally shaping how decisions are made regarding patient care. In an era where evidence-based practice is king, understanding clinical outcomes allows for more informed decisions concerning treatments and interventions. The significance lies not merely in numbers on a chart but in the stories these figures tell about patient experiences and the overall effectiveness of care delivered.
For instance, knowing the rates of recovery or incidence of complications after a specific procedure offers healthcare providers the leverage to weigh risks against benefits efficiently. This discernible influence on treatment decisions is crucial, acting as a guide in steering both patients and practitioners towards pathways that maximize benefits while minimizing adverse effects.
A closer look reveals a few key areas influenced by this measurement:
- Improved Resource Allocation: Assessing outcomes helps hospitals and clinics allocate resources effectively. For example, if data reveals that a particular treatment option leads to significantly higher recovery rates, more funding and training can be directed towards that option.
- Informed Consent: Patients gain a clearer understanding of what to expect from treatments, leading to more informed consent processes. When discussing procedures, practitioners can reference specific outcome data, enabling patients to feel more confident in their choices.
- Quality Improvement Initiatives: Systematic outcome measurement promotes a culture of constant improvement. Hospitals can identify areas that lag behind, focusing efforts on enhancing those to ensure a standard of care that meets the highest benchmarks.
"Data-driven care isn't just about trendsāit's about transforming lives. Understanding the impact we have through measurements spurs us toward better outcomes."
Additionally, there's a notable shift towards personalized medicine. This individualized approach responds back to measurement techniques, prioritizing the unique genetic and environmental factors influencing patient health. Tailored treatment strategies are being designed based on how specific groups respond to different interventions, marking a significant departure from one-size-fits-all methods.
Influence on Treatment Decisions
The influence of clinical outcome measurement on treatment decisions cannot be overstated. It serves not just as a tool for assessment but also as a framework guiding healthcare providers in their choices. For instance, when hospitals observe higher survival rates associated with a specific surgical procedure compared to alternative methods, the implications are enormous. Surgeons and medical teams can lean towards the better-performing options, which elevates overall patient care quality.
Moreover, by analyzing outcomes, healthcare professionals can identify patterns and correlations that would otherwise go unnoticed. If a certain demographic consistently fares worse under standard treatments, nuanced adjustments can be made to tailor interventions that meet their needs more effectively.

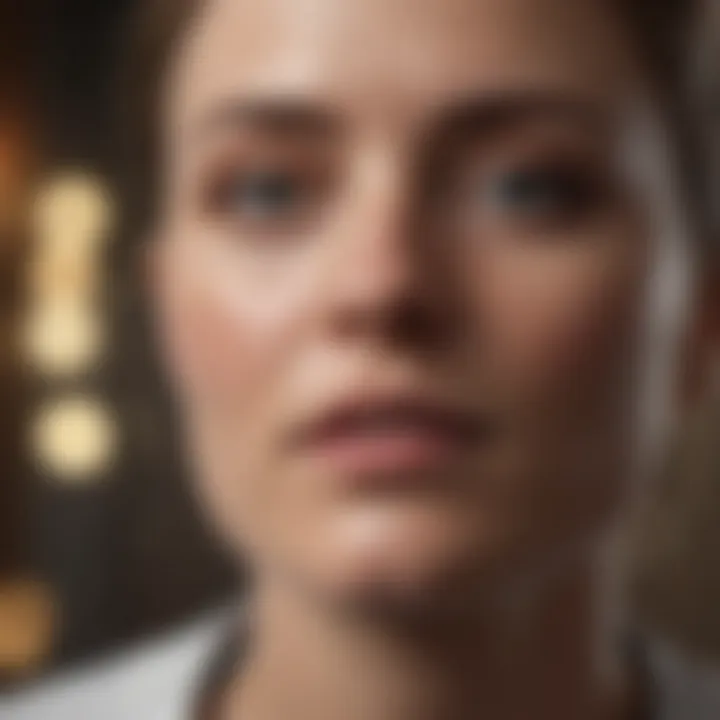
- Real-Time Adjustments: Continuous outcome monitoring allows for on-the-fly adjustments during treatment. For example, should initial treatment prove ineffective, real-time data can signal a switch to an alternative approach without delay.
- Benchmarking Against Best Practices: Facilities often benchmark their outcomes against national or international standards. This comparison helps in striving for excellence, motivating teams to match or exceed top-notch care levels.
Personalized Medicine and Tailored Treatments
The future of healthcare seems set on the road of personalization, where treatments resonate more closely with individual patient profiles. The utilization of outcome measurement is a cornerstone of this movement. Personalized medicine, which hinges on understanding the variation in individuals' responses to treatment based on genetic, environmental, and lifestyle factors, is a promising route.
- Data-Driven Profiles: Clinicians can create detailed patient profiles using historical outcome data. If a patient with a specific genetic marker shows a pattern of positive response to a medication, that medication can become the first line of treatment.
- Enhanced Efficacy: With tailored treatment plans, patients are likely to experience improved efficacy in their care. Reduced trial and error means less time wasted on ineffective therapies.
Ultimately, measuring clinical outcomes fosters a landscape where precision in interventions is prioritized. Medical professionals stand to gain valuable insights from the data available, enabling them to forge treatment plans that address the needs of not just the majority, but the individual, thereby significantly enhancing overall patient satisfaction and health outcomes.
Ethics and Challenges in Measuring Outcomes
The landscape of clinical outcome measurement is not just a complex terrain of data and statistics; it is also a field interwoven with ethical considerations and challenges that impact how outcomes are measured and interpreted. Grasping this aspect is paramount, as ethical dilemmas can shape the validity of results and ultimately affect patient care. Additionally, understanding the barriers that exist in accurate measurement highlights the intricacies that healthcare professionals must navigate. Considering these issues creates a more comprehensive view of clinical outcomes and informs the practices within healthcare settings.
Ethical Considerations
Ethical considerations in measuring clinical outcomes encompass a range of practices that must be carefully evaluated to protect patient rights and ensure integrity in data collection. Adhering strictly to ethical guidelines is crucial, especially when working with sensitive patient information. Here are some key elements:
- Informed Consent: Patients should be fully aware of their participation in studies, including what their data will be used for. They must understand the potential risks and benefits involved.
- Confidentiality: Safeguarding patient information is non-negotiable. Any data that could trace back to an individual needs to be anonymized to maintain privacy.
- Bias Avoidance: Researchers must strive to eliminate biases in outcome measurement. This includes biases in study design, data interpretation, and reporting outcomes.
- Representativeness: Ensuring diverse populations are included in studies helps avoid skewed results that could favor certain demographics over others.
Ethical lapses in clinical research can lead to loss of public trust and hinder medical advancement. The ripple effect can touch every corner of healthcare, making adherence to ethics non-negotiable.
The ethical landscape also extends to the dissemination of results. How findings are communicated can greatly influence public perception and patient decision-making, presenting yet another layer of responsibility for researchers and clinicians alike.
Barriers to Accurate Measurement
When it comes to accurately measuring clinical outcomes, various barriers can hinder progress and skew results. Understanding these limitations is essential for future improvements in measurement practices and patient care.
- Variability in Patient Responses: Each patient responds differently to treatments due to factors like genetics, environment, and lifestyle. This variability makes it challenging to create standardized outcomes that apply universally.
- Data Quality Issues: Problems such as incomplete records, errors in data entry, and lack of standardized definitions for outcomes can compromise the accuracy of measurements.
- Resource Constraints: Not every healthcare facility has the capacity to implement robust data collection systems. Limitations in funding, staffing, and technology can further exacerbate this issue.
- Patient Engagement: Engaging patients in the process of outcome measurement is vital, yet many patients may not have the time or incentive to actively participate. Without their involvement, data can become sparse or biased.
- Technological Limitations: While technological advances can enhance data collection, they can also introduce complexities. Issues such as interoperability between systems can hinder the seamless exchange of information necessary for accurate measurement.
Addressing these barriers requires a multi-faceted approach that includes education, improved technologies, and systemic changes within healthcare organizations. This ongoing evolution will not only advance the field of clinical outcomes measurement but also significantly benefit patient care.
The Future of Clinical Outcome Measurement
The future of clinical outcome measurement holds significant promise for transforming the healthcare landscape. With a keen focus on enhancing patient experiences and treatment effectiveness, ongoing advancements are set to address existing challenges and pave the path for innovative solutions. The incorporation of new technologies, data sources, and methodologies ensures that healthcare professionals can engage in evidence-based practices backed by robust clinical outcomes.
As the field evolves, stakeholders must also consider the implications of these changes. Ethical considerations come into play, particularly when leveraging advanced technologies for outcome measurement. Nevertheless, the benefits far outweigh the challenges, making the exploration of these frontiers both crucial and timely.
Emerging Technologies
The impact of emerging technologies on clinical outcome measurement cannot be overstated. Consider how telemedicine, wearable devices, and mobile health apps are currently reshaping the patient monitoring process. For instance, wearables like Fitbit or Apple Watch collect real-time data on heart rates, physical activity, and sleep patterns, offering invaluable insights into a patient's health status. Doctors can use this data for continuous monitoring and early intervention, leading to improved outcomes.
Moreover, innovations such as blockchain technology emerge to address data management issues, ensuring the security and transparency of sensitive health information. By facilitating better data sharing among healthcare providers, the goals of interoperability and comprehensive patient care become more attainable.
- Potential areas of impact include:
- Reduced hospital readmissions
- Improved patient adherence to treatment plans
- Enhanced precision in managing chronic diseases
These advancements can ultimately lead to tailored therapies that suit individual patient needs, emphasizing preventive care and quality of life over traditional metrics.
Integration of Big Data and AI
As healthcare systems generate vast amounts of data, the integration of big data analytics and artificial intelligence (AI) stands to revolutionize clinical outcome measurement. These technologies enable healthcare professionals to analyze complex datasets quickly, delivering insights that were previously unimaginable.
AI algorithms can sift through historical patient data, identifying patterns and predicting potential outcomes for various treatment options. For example, machine learning models can help decide the most effective interventions for patients with similar health profiles, thereby fostering a more personalized approach to care.
"Incorporating AI into clinical outcome measurement enhances our ability to track and interpret data efficiently, making strides toward precision medicine."
Additionally, big data analytics can aid in the identification of social determinants of health, revealing how factors such as socioeconomic status or geographic location influence treatment effectiveness. This comprehensive understanding of patient contexts allows for more informed and equitable healthcare delivery.
- Key advantages of this integration include:
- Enhanced predictive modeling for outcomes
- Efficient resource allocation in treatment plans
- Improved quality of care through data-driven decisions
Finale
Measuring clinical outcomes is not merely a procedural formality; it's a cornerstone in the pursuit of effective healthcare. The conclusion of this comprehensive investigation highlights several key elements concerning the measurement of clinical outcomes which are vital for both practitioners and stakeholders in the healthcare arena.
One essential consideration is the understanding of the significance behind accurate outcome measurement. By thoroughly assessing treatment interventions, healthcare providers can make informed decisions that do not only consider immediate effects but long-term patient well-being as well. This goes beyond numbers; itās about real lives affected by those outcomes. It's where clinical efficacy meets the realm of human experience.
Summary of Key Points
- Improved Patient Care: Each aspect of clinical outcome measurement translates into tangible benefits for patient health. When outcomes are accurately measured, it leads to more personalized and effective treatments.
- Enhanced Decision-Making: Clinicians and health organizations can utilize data to refine strategies, leading to better allocation of resources.
- Identification of Best Practices: By observing what works through empirical data, healthcare systems can continually adapt and hone their approaches.
- Ethical Responsibility: Thereās a profound moral obligation to use outcome data responsibly to advocate for patients' needs within the healthcare system.
"The true measure of healthcare success is found not just in treatment but in outcomes that resonate with the patients' lived experiences."
The Path Forward in Clinical Outcome Measurement
Looking ahead, the journey towards refining clinical outcome measurement is filled with opportunities. Emerging technologies like telemedicine, and mobile health applications are set to revolutionize how we gather and analyze data. These innovations not only facilitate greater data collection but enable real-time monitoring of patient conditions.
Furthermore, the integration of big data and artificial intelligence into healthcare promises to enhance predictive analytics and personalize care even more effectively. By harnessing vast datasets, practitioners can uncover patterns and trends that manual methods might overlook.
- It's also crucial to engage patients in the measurement process, ensuring that their voices shape outcome definitions.
- Moreover, collaboration across multidisciplinary teams will be essential in ensuring a holistic approach and addressing the multifaceted nature of health outcomes.
In summary, moving forward in measuring clinical outcomes requires a blend of technology, collaboration, and an unwavering commitment to patient-centric care. The focus should always be on how these measurements ultimately enrich the patient experience and elevate standards of care in an ever-evolving healthcare landscape.