Understanding Biomedical Informatics in Healthcare
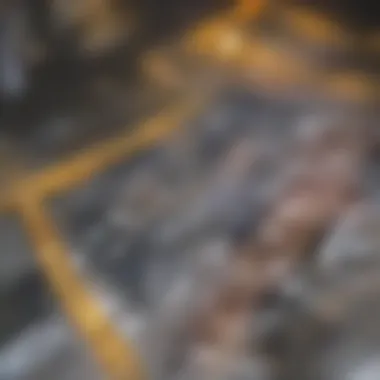
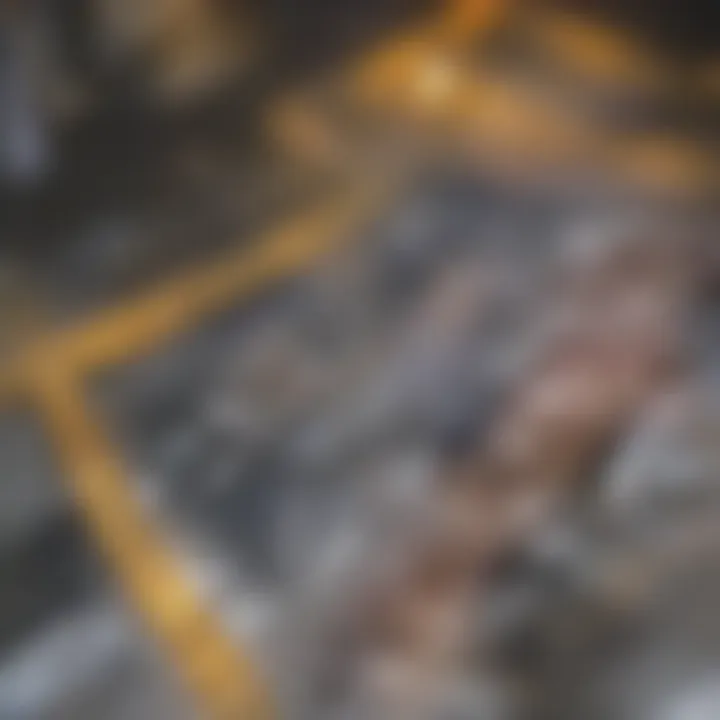
Intro
Biomedical informatics is an interdisciplinary field that plays a vital role in modern healthcare. It combines various domains, such as computer science, information science, and healthcare itself, to enhance the management of medical data and improve patient care outcomes. Understanding the key concepts and terminology related to this field is essential for anyone looking to dive deeper into its applications and implications.
The significance of biomedical informatics can be observed in its ability to bridge the gap between technology and healthcare. As we continue to generate vast amounts of data in medical settings, effective management and analysis become imperative. This article explores the fundamental components of biomedical informatics, its practical applications, the challenges it faces, and the potential for future developments.
Key Concepts and Terminology
Definition of Key Terms
In biomedical informatics, several core terms are essential for a comprehensive understanding:
- Electronic Health Records (EHRs): Digital representations of patient health data. EHRs facilitate easy access to patient information and enable improved care coordination among healthcare providers.
- Health Information Exchange (HIE): A system that allows healthcare information to be shared across organizations and professionals, promoting a holistic view of patient health.
- Clinical Decision Support Systems (CDSS): Computer systems designed to assist healthcare providers in making informed decisions based on patient data, clinical guidelines, and relevant research.
- Big Data: Large and complex data sets that traditional data processing applications cannot manage effectively. In healthcare, big data analytics can lead to improved patient outcomes and operational efficiency.
Concepts Explored in the Article
This article delves into the following concepts:
- The role of data management in enhancing patient care.
- The integration of machine learning and artificial intelligence in predictive analytics for better diagnosis and treatment options.
- The implications of telemedicine and remote monitoring for expanding access to care, especially in underserved areas.
Findings and Discussion
Main Findings
The exploration of biomedical informatics reveals several key findings:
- Effective management of health data can lead to significant improvements in patient outcomes.
- The use of predictive analytics can enhance decision-making for healthcare professionals, leading to timely interventions.
- Interoperability among diverse health information systems remains a critical challenge, impacting the quality of care delivery.
"The effective integration of technology in healthcare can transform patient experiences and outcomes."
Potential Areas for Future Research
Research in biomedical informatics has several trajectories that could yield fruitful insights:
- Enhancing data security: As patient data digitizes, ensuring privacy and compliance with regulations is crucial.
- Developing more robust predictive analytics tools: These tools can potentially revolutionize how conditions are diagnosed and treated.
- Improving user interfaces: Streamlining the usability of health information systems for better adoption by medical professionals is essential for widespread success.
Through this exploration, it becomes evident that biomedical informatics is not only a field of study but a critical driver of innovations and improvements in healthcare. Understanding its key concepts, applications, and future directions will help enthusiasts and professionals alike contribute meaningfully to its evolution.
Preface to Biomedical Informatics
Biomedical informatics is positioned at the intersection of technology and healthcare, fundamentally transforming how we manage and utilize medical data. This discipline leverages principles from computer science, information science, and health sciences to enhance patient care and medical research. The significance of biomedical informatics cannot be overstated; it underpins many modern healthcare initiatives aiming for greater efficiency and improved outcomes.
Definition and Scope
Biomedical informatics can be defined as a field that integrates various aspects of computing with biomedicine. It encompasses a wide range of activities, including the collection, storage, analysis, and dissemination of biomedical data. The scope of this field is vast, involving:
- Data Management: Systematic organization of health data, such as patient records, clinical trials, and research findings.
- Knowledge Representation: Methods for encoding medical knowledge to facilitate understanding and interoperability.
- Information Retrieval: Techniques for efficiently accessing relevant medical literature and data.
This field is crucial for enhancing decision-making processes in clinical environments and fostering innovation in biomedical research. A common misconception is that biomedical informatics solely focuses on technology. However, its success relies heavily on understanding the context of healthcare and the needs of patients and practitioners.
Historical Context
To appreciate the current advancements in biomedical informatics, one must consider its historical context. The emergence of electronic health records in the 1960s marked a pivotal moment. Initially intended to improve record-keeping, these systems evolved alongside computing technology.
The 1990s saw significant growth in the field, with the establishment of dedicated research centers and the introduction of standardized formats for health information. Subsequently, the rise of the internet has catalyzed the development of web-based healthcare solutions, making data more accessible than ever.
Notably, the Human Genome Project, completed in the early 2000s, demonstrated the power of informatics in managing vast genomic data. This monumental initiative paved the way for new research methodologies and clinical applications.
In summary, the evolution of biomedical informatics reflects an ongoing commitment to integrating complex data systems into the healthcare landscape. Understanding this history helps professionals recognize the roots of current practices and the trajectory toward future innovations.
"Biomedical informatics is not just about technology; it is about meaningful interaction between data, knowledge, and healthcare professionals."
This introduction lays the groundwork for further exploration into the core components, applications, and ethical considerations that define biomedical informatics today.
Core Components of Biomedical Informatics
Biomedical informatics relies on several core components to ensure effective management of healthcare data. These components, which encompass data management, knowledge representation, and information retrieval, are critical for making informed decisions in clinical practice and research. Understanding these elements is essential for students, researchers, and professionals in the field, as they define how data is collected, interpreted, and utilized to enhance patient care.
Data Management
Data management is the process of acquiring, storing, and organizing healthcare data in a way that ensures its accuracy and availability. Effective data management supports the integration of diverse data types, such as clinical, operational, and demographic data, which is important for improving healthcare delivery. The importance of data management cannot be overstated, as it influences both the quality of information available for decision-making and the efficiency of healthcare systems.
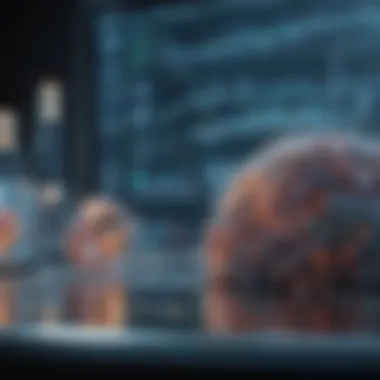
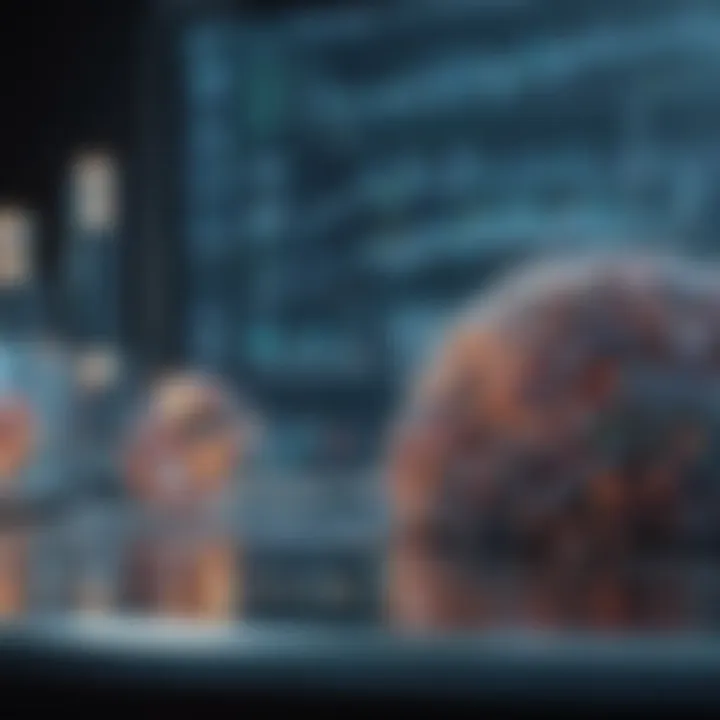
To manage data effectively, healthcare organizations often utilize a range of tools and technologies, such as databases, electronic health records (EHRs), and data warehouses. These systems facilitate the secure storage and retrieval of data while allowing for real-time access by healthcare providers. Additionally, robust data management practices help in meeting regulatory compliance and ensuring patient privacy.
Knowledge Representation
Knowledge representation in biomedical informatics refers to the methods used to encode and structure medical knowledge so that it can be easily accessed and processed by computer systems. This component is vital in creating systems that support clinical decision-making. Knowledge representation often involves ontologies, which are frameworks that define the concepts and relationships within a specific domain, such as medicine.
By using standardized terminologies, like SNOMED CT or LOINC, clinical data can be shared and interpreted consistently across different systems. Knowledge representation supports the development of clinical decision support systems (CDSS) that assist clinicians in diagnosing and treating patients based on evidence-based guidelines. Moreover, effective knowledge representation reduces variability in care, leading to improved patient outcomes.
Information Retrieval
Information retrieval is the process of obtaining relevant data and knowledge from large databases and information sources. In the context of biomedical informatics, it helps healthcare professionals access critical information quickly, facilitating timely decision-making. Information retrieval systems are designed to help users search through vast amounts of data, including medical literature, patient records, and clinical trial databases.
Search engines used in health informatics often employ advanced algorithms to match queries with relevant results. They utilize techniques from natural language processing and machine learning to improve the efficiency and accuracy of searches. Effective information retrieval not only aids clinicians in finding information but also plays a role in research by allowing scientists to identify trends and insights from existing data.
In summary, the core components of biomedical informatics — data management, knowledge representation, and information retrieval — play a crucial role in shaping modern healthcare. Together, they facilitate the efficient use of medical data, enhance clinical decision-making, and improve patient outcomes. Understanding these components is integral for anyone looking to navigate the complex intersection of information technology and healthcare.
Applications in Healthcare
Applications in healthcare represent a pivotal aspect of biomedical informatics. Recognizing this importance allows us to appreciate how technological advancements shape patient care and enhance the healthcare system overall. In this section, we will analyze key applications, including clinical decision support systems, electronic health records, telemedicine, and data mining. Each component contributes uniquely to better health outcomes and operational efficiency.
Clinical Decision Support Systems
Clinical Decision Support Systems (CDSS) are tools that provide healthcare professionals with knowledge and patient-specific information to assist in decision-making. These systems analyze data from various sources, including electronic health records and clinical guidelines, to deliver real-time recommendations. The implementation of CDSS results in improved adherence to best practices and minimized medication errors.
Some benefits of CDSS include:
- Improved patient safety: Reducing potential adverse drug interactions by alerting providers.
- Enhanced efficiency: Streamlined workflow by offering standardized recommendations.
- Evidence-based support: Leveraging research data to guide clinical decision-making.
Despite their advantages, CDSS comes with considerations as well. Providers might experience information overload or mistrust in system recommendations. Consequently, it is crucial to design user-friendly interfaces that enhance user acceptance and trust in the system.
Electronic Health Records
Electronic Health Records (EHRs) are digital versions of patients' medical histories. They serve as a comprehensive source of information, including demographics, diagnoses, medications, and treatment plans. EHRs facilitate easy access to patient data, which enhances communication among healthcare providers.
The importance of EHRs can be highlighted through several factors:
- Comprehensive data access: Physicians gain a holistic view of patient health across different care settings.
- Improved coordination: Enhanced communication among healthcare teams reduces redundancies and errors.
- Data for research: EHRs collect large datasets that drive research and quality improvement initiatives.
However, challenges exist, such as maintaining data security and ensuring usability for different healthcare providers.
Telemedicine and Remote Monitoring
Telemedicine has gained significant traction, especially in the context of global health crises. By utilizing telecommunications technology, patients can receive care remotely, thus overcoming geographical barriers. This method not only improves access to healthcare but also fosters timely intervention.
Key features include:
- Accessibility: Patients in rural areas can consult with specialists without traveling long distances.
- Convenience: Flexible appointment scheduling allows for ease of access.
- Continuous patient monitoring: Wearable devices gather health data for ongoing patient assessment.
While telemedicine holds great promise, concerns related to data privacy and the quality of care delivery persist. Policymakers must address these issues to maximize benefits while safeguarding patient information.
Data Mining and Predictive Analytics
Data mining and predictive analytics are techniques that analyze vast amounts of healthcare data to identify trends and predict patient outcomes. Utilizing advanced algorithms, these technologies transform raw data into actionable insights. This is particularly crucial in predicting potential health issues before they escalate.
Advantages include:
- Trend identification: Continuous monitoring of population health can reveal emerging diseases or conditions.
- Resource allocation: Predictive models assist healthcare administrators in planning resource allocation effectively.
- Personalized treatment: Insights can help customize treatment plans tailored to individual patient needs.
The ethical implications of data mining in health care also need discussion. It is essential to balance the benefits of data insights against issues of privacy and consent.
In summary, the applications of biomedical informatics in healthcare not only improve patient care but also enhance operational efficiencies. Whether through supporting clinical decisions, providing comprehensive health records, facilitating remote consultations, or leveraging predictive analytics, these applications redefine modern healthcare.
These tools exemplify how technology plays an integral role in the ongoing evolution of healthcare. The rich interplay of these elements will continue to shape the future of patient care, ensuring that healthcare delivery is both efficient and patient-centered.
Research and Development in Biomedical Informatics
Biomedical informatics is a crucial field for advancing healthcare through innovative technologies. Research and development (R&D) in this area drives the creation of tools and methodologies that support patient care, streamline data management, and foster discoveries in biomedicine. As the landscape of healthcare continues to evolve, R&D in biomedical informatics plays an indispensable role in addressing contemporary challenges, making it pertinent to explore its various dimensions.
Translational Bioinformatics
Translational bioinformatics serves as a bridge between laboratory discoveries and clinical applications. It enables researchers to convert biological data into meaningful insights that can affect patient care positively. The integration of genomic data into clinical practice is one of the most notable aspects of translational bioinformatics. This integration supports the identification of biomarkers and the development of targeted therapies, ultimately leading to more personalized treatment plans.
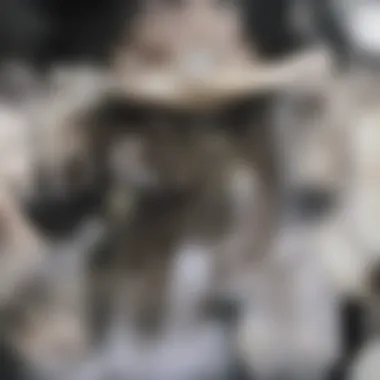
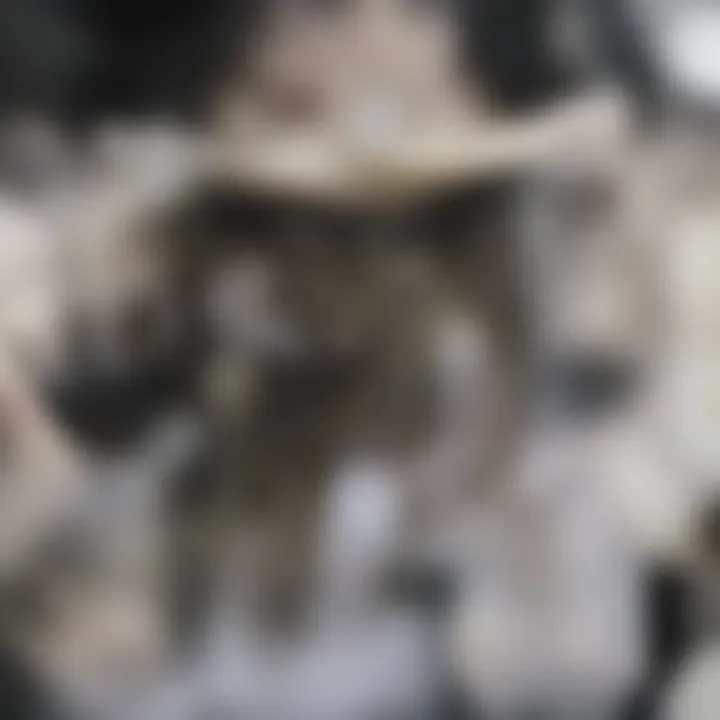
Translational bioinformatics relies heavily on computational tools to analyze large datasets. This includes data from genomics, proteomics, and metabolomics. Effective management and interpretation of this data can potentially uncover trends and correlations that were previously overlooked. The advancements in machine learning contribute significantly to this area by enhancing predictive models based on these vast data sets.
Genomics and Biomedical Data
Genomics is a pivotal component of biomedical informatics, particularly as the volume of genomic data continues to increase. The rise of next-generation sequencing technologies has generated massive amounts of data that must be analyzed and interpreted. This flood of information presents both opportunities and challenges for healthcare professionals and researchers.
Incorporating genomic data into healthcare can lead to significant advancements in diagnostics and treatment. For instance, healthcare providers can make informed decisions based on a patient's genetic profile. This customized approach is termed precision medicine, which aims to tailor interventions to individual characteristics, needs, and preferences.
Furthermore, bioinformatics tools that facilitate the analysis of genomic data are essential for advances in medical research and clinical practice. They help in identifying genetic mutations, understand disease mechanisms, and find potential therapeutic targets. Developing robust genomic databases is vital for supporting researchers and practitioners in this domain.
Clinical Research Informatics
Clinical research informatics focuses on integrating information technology with clinical research, enhancing the quality and efficiency of clinical trials. It facilitates the collection, management, and analysis of data generated from clinical studies. By leveraging electronic data capture systems and databases, researchers can document research protocols, patient outcomes, and adverse events effectively.
Key aspects of clinical research informatics include:
- Data integration: Combining data from different sources enables more comprehensive analysis and helps identify patterns.
- Regulatory compliance: Effective informatics tools ensure that clinical research adheres to regulatory requirements and maintains data integrity.
- Collaboration: Enhancing communication between various stakeholders—researchers, clinicians, and patients—facilitates smoother trial processes and improves outcomes.
The evolution of clinical research informatics is critical in fostering innovation within biomedicine. It streamlines processes and ensures that newly generated knowledge effectively influences clinical practice, which can lead to improved patient care.
"Research and development in biomedical informatics not only supports innovation but also enhances understanding of complex biological processes, impacting healthcare on multiple levels."
Interdisciplinary Nature of Biomedical Informatics
Biomedical informatics stands at the crossroads of various fields, facilitating a comprehensive approach to healthcare challenges. Understanding its interdisciplinary nature is vital for appreciating how it optimally serves modern medicine. Its core lies in the collaboration between multiple domains including computer science, health sciences, and behavioral sciences. Each discipline contributes unique perspectives and skills, fostering innovation and improved patient outcomes.
Collaboration with Computer Science
The collaboration with computer science is central to the efficacy of biomedical informatics. Computer science provides the tools and techniques necessary for analyzing large datasets, developing algorithms, and creating user-friendly interfaces for healthcare professionals. In particular, advanced computational methods allow for better management of electronic health records, enabling seamless access to patient information across different platforms.
Moreover, the implementations of machine learning algorithms enhance predictive analytics protocols. These innovations assist clinical decision support systems, ensuring healthcare providers have accurate and timely information at their fingertips. This partnership significantly streamlines processes, reducing errors and improving the quality of care.
Integration with Health Sciences
Integration with health sciences further enriches the field of biomedical informatics. By closely working with experts in clinical practices and health policy, biomedical informatics professionals can devise solutions tailored to specific medical needs. This collaboration allows for a deeper understanding of healthcare delivery systems, which is essential for designing effective informatic interventions.
Coordinated efforts in this realm help align technological solutions with the realities of patient care. For example, developing robust electronic health records requires input from clinicians to ensure that the data captured are relevant and usable for ongoing patient management. This dialogue bridges gaps between theory and practice, enabling more effective healthcare solutions.
Influence of Behavioral Sciences
The influence of behavioral sciences in biomedical informatics is often overlooked but remains critical. Understanding patient behavior and clinician decision-making shapes the way informatics tools are designed. Insights from psychology and sociology inform strategies on how to encourage patient engagement and adherence to treatment plans. Furthermore, analyzing user experience in healthcare software allows developers to create systems that align with human behaviors and preferences.
By incorporating elements from behavioral science, biomedical informatics can promote better health literacy among patients, ultimately leading to improved therapeutic outcomes. This holistic approach ensures that informatics applications address not just data management, but also the psychological and social dimensions of health behavior.
"Interdisciplinary collaboration is key to addressing the complexities of modern healthcare challenges, where technology meets human needs."
Ethical Considerations in Biomedical Informatics
Ethical considerations in biomedical informatics are critical for ensuring that the integration of technology in healthcare serves the best interests of all stakeholders. The intersection of healthcare and information technology creates complex dilemmas and responsibilities. This section discusses three areas of primary concern: patient privacy and data security, bias and fairness in algorithms, and informed consent in data use.
Patient Privacy and Data Security
The protection of patient privacy is a cornerstone in biomedical informatics. As vast amounts of sensitive health information are collected, it is imperative to implement robust data security measures. Any breach may not only lead to personal harm to individuals if their medical data is exposed but could erode public trust in healthcare systems.
Organizations must adhere to regulations such as HIPAA (Health Insurance Portability and Accountability Act) to safeguard patient information. Encryption and access controls are technical safeguards that help protect data. Additionally, ethical policies need to ensure that data usage is transparent and that patients are informed about how their data is handled.
"In the digital age, safeguarding patient privacy is not just a legal requirement, but an ethical obligation that impacts trust and healing in healthcare."
Bias and Fairness in Algorithms
Algorithms are increasingly being utilized to inform clinical decisions. However, the algorithms are only as good as the data fed into them. If the data are biased, the results will also be biased. This raises questions about fairness in treatment recommendations and healthcare access.
It’s essential to strive for diverse datasets that reflect the demographic variations of the population. Addressing potential biases requires a conscious effort to review algorithm performance across different groups. Continuous monitoring and adjustment of algorithms can help mitigate biases, ensuring that all patients receive equitable treatment.
Informed Consent in Data Use
Informed consent is a fundamental principle in biomedical ethics. Patients need to understand not only what data is being collected, but also how it will be used. Transparency is key to fostering trust. Patients should be educated about the implications of their data being utilized for research or analysis.
Instituting clear and user-friendly consent processes is imperative. Forms should avoid complex jargon and clearly outline the scope of data use. Patients must feel empowered to make decisions regarding their data without coercion.
In summary, the ethical considerations in biomedical informatics are multifaceted and significant. Addressing issues of privacy, bias, and consent will contribute to the effective and ethical deployment of informatics in healthcare, ensuring that it serves the needs of all involved.
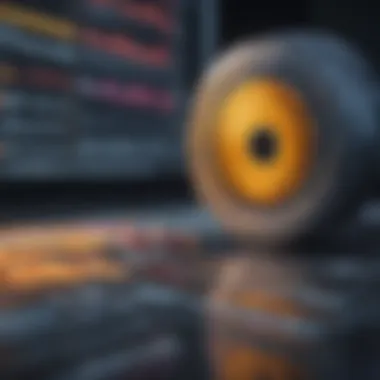
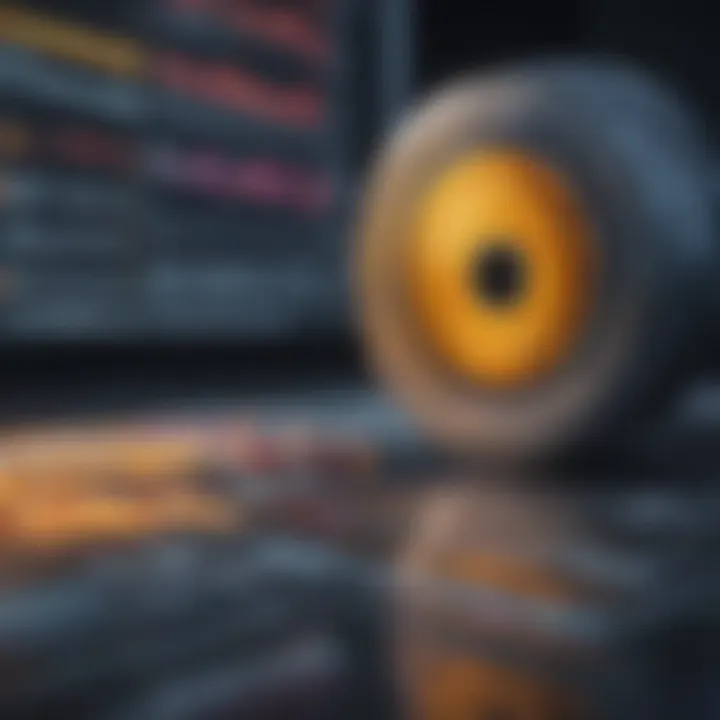
Challenges in Implementing Biomedical Informatics
Implementing biomedical informatics comes with a range of challenges. Identifying these challenges is vital for medical professionals, researchers, and technologists. Proper understanding can lead to effective solutions and better healthcare outcomes. This section discusses major challenges, focusing on interoperability issues and scalability of systems.
Interoperability Issues
Interoperability is crucial for effective communication between different health information systems. It allows for the seamless exchange of data among various healthcare providers. Without interoperability, patient data may be trapped in siloed systems, leading to inefficiencies and potential gaps in patient care. The ability to share clinical data across platforms can result in enhanced patient safety, improved outcomes, and reduced costs.
Consider the following factors when addressing interoperability:
- Standardization of Data: Adopting common data standards improves communication. Standards such as Health Level Seven (HL7) or Fast Healthcare Interoperability Resources (FHIR) play significant roles.
- Technology Infrastructure: Organizations need to build or upgrade infrastructure that supports data sharing. This requires both technical and financial resources.
- Collaboration Among Stakeholders: Interoperability often requires collaboration among different healthcare entities. Trust and mutual agreements must be established among stakeholders.
Interoperability issues are not just technical. They invoke organizational policies and workflows that can either hinder or promote data sharing.
Scalability of Systems
Scalability refers to a system's ability to handle growing amounts of work or its potential to accommodate growth. In biomedical informatics, as patient data continues to escalate, systems must be able to expand efficiently. If a system cannot scale effectively, it may lead to performance bottlenecks or even data loss.
Key considerations include:
- Flexible Architecture: Systems should use adaptable architectures that can respond to changing needs. Cloud-based solutions often offer better scalability compared to traditional on-premise solutions.
- Load Management: Equipping systems to balance loads will help manage increased traffic without sacrificing performance.
- Investment in Technology: Continuous investment in newer technologies is paramount. This allows healthcare providers to leverage the latest advancements.
The scalability challenge is not only about technology but also about organizational readiness. Employees need to be trained to handle new tools and systems that support scalability.
In summary, addressing interoperability and scalability is essential in overcoming challenges within biomedical informatics. Successfully navigating these challenges lays the groundwork for future advancements and greater efficiency in healthcare delivery.
Future Directions in Biomedical Informatics
The field of biomedical informatics is dynamic and rapidly evolving. As technology advances, it brings with it new opportunities and challenges that impact healthcare delivery, research, and patient outcomes. Understanding future directions in this domain is essential, as it helps stakeholders in healthcare navigate the complexities surrounding data management and innovation. Emerging technologies play a pivotal role in shaping healthcare, making it crucial to explore the implications, benefits, and ethical considerations involved in these innovations.
Artificial Intelligence and Machine Learning
Artificial Intelligence (AI) and Machine Learning (ML) are at the forefront of biomedical informatics. These technologies analyze vast amounts of data to uncover patterns and insights that human analysts might miss. For example, AI algorithms can predict patient deterioration by examining electronic health records, enhancing early intervention strategies.
The potential applications are extensive:
- Diagnostic Tools: AI assists in interpreting medical images, such as MRIs and CT scans, improving accuracy.
- Treatment Personalization: Machine learning models can identify optimal treatment plans based on individual patient data, increasing the likelihood of favorable outcomes.
- Predictive Analytics: Hospitals can use predictive models to manage resources more effectively by anticipating higher patient volumes during flu seasons.
However, the implementation of AI and ML must be approached carefully. Issues surrounding data quality, bias in algorithms, and the transparency of decision-making processes require attention.
Blockchain Technology in Healthcare
Blockchain technology offers a promising framework for managing medical records securely and transparently. Its decentralized nature enhances data integrity, which is vital for protecting patient privacy and fostering trust. Through blockchain, patients can control access to their own data, allowing them to share necessary information with healthcare providers while maintaining confidentiality.
Key benefits of applying blockchain in healthcare encompass:
- Security: Cryptographic techniques make it significantly harder for data breaches to occur.
- Interoperability: Blockchain systems can facilitate seamless data exchange among different healthcare providers.
- Audit Trails: Immutable logs can help track access and changes to patient data, ensuring accountability.
Yet, challenges remain in terms of scalability and regulatory compliance. Collaboration between technology experts, healthcare providers, and policy makers will be essential to overcome these obstacles.
Personalized Medicine and Informatics
Personalized medicine relies heavily on informatics to tailor medical treatments to individual patients based on their genetic, environmental, and lifestyle factors. This approach moves away from the one-size-fits-all model of treatment. Instead, it harnesses data to delineate specific patient needs, thus enhancing the efficacy of therapeutic interventions.
Several aspects highlight its significance:
- Genomic Data Utilization: Insights from genomics can guide the development of individualized medications.
- Patient Engagement: Informatic tools can provide patients with tailored health information, enabling them to make informed decisions about their care.
- Outcomes Measurement: By analyzing treatment responses on an individual level, healthcare providers can refine treatment pathways.
While the promise of personalized medicine is significant, it poses substantial challenges. Ethical considerations surrounding genetic data and the societal implications of data-driven healthcare must be carefully examined.
"The integration of advanced technologies in biomedical informatics paves the way for a future where healthcare is more efficient, personalized, and secure, ultimately improving patient care and outcomes."
As we look forward, it is clear that embracing innovative technologies such as AI, blockchain, and personalized medicine will shape the future landscape of biomedical informatics. The evolution in this field demands a commitment to staying informed and addressing ethical concerns, making it crucial for all stakeholders to collaborate in finding effective solutions.
Epilogue
The conclusion of this article serves as a pivotal reflection on the importance of biomedical informatics in contemporary healthcare. This field not only integrates computer science with healthcare but also revolutionizes how we manage and interpret medical data.
Through a thorough exploration of the various elements outlined in this article, it becomes evident that biomedical informatics holds significant benefits for practitioners, researchers, and patients alike. Its core components—ranging from data management to predictive analytics—enhance clinical workflows and support better decision-making.
Considering the challenges posed by interoperability and system scalability, the field's evolution is not without complexities. However, addressing these issues lays the groundwork for impactful advancements. Moreover, the intricate relationship between technology and healthcare opens-up pathways for innovative solutions like personalized medicine and AI applications.
In summary, the synthesis of ideas presented throughout this article emphasizes that biomedical informatics is not just a tool, but a necessary force that drives development in patient care and public health. As we move forward, the continuous adaptation of these informatic methods will play a critical role in shaping the future of healthcare.
"The integration of technology in healthcare positions biomedical informatics as an essential discipline for improving patient outcomes and advancing research."
Recognizing the relevance of this field is essential for anyone involved in healthcare. Their engagement with biomedical informatics can lead to enhanced outcomes that are both measurable and meaningful.