Understanding Predictive Demand: Insights & Impacts
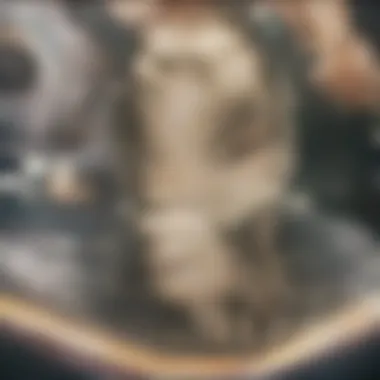
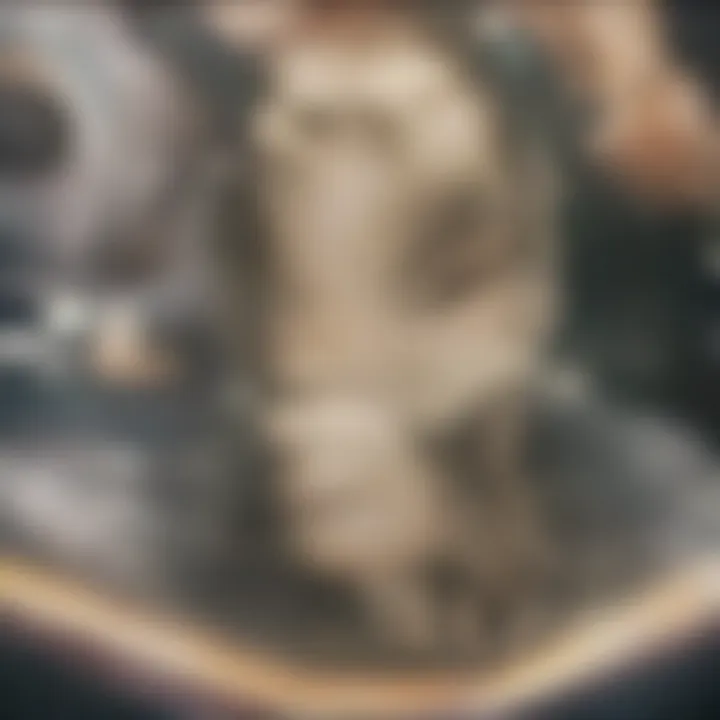
Intro
Predictive demand has emerged as a vital component in navigating a world that increasingly relies on data to drive decisions. The significance of accurately forecasting demand cannot be overstated, especially in sectors like supply chain management and marketing. As organizations strive to optimize their operations, understanding predictive demand becomes essential for maintaining competitiveness and relevance.
This article aims to dissect the concept of predictive demand, shedding light on the methodologies and technologies that underpin effective demand forecasting. Data analytics, machine learning, and consumer behavior analysis play pivotal roles in this arena, providing insights that enhance predictive accuracy. Through a careful examination of various industry applications, we will identify challenges and ethical considerations that accompany the use of data. Ultimately, the goal is to furnish a comprehensive understanding of predictive demand in today’s data-driven landscape.
Understanding Predictive Demand
Understanding predictive demand is crucial in today’s data-centric environment. The ability to anticipate consumer needs not only facilitates better inventory management but also optimizes supply chain processes. Organizations that grasp predictive demand can tailor their strategies effectively, leading to enhanced customer satisfaction and increased profitability.
Definition of Predictive Demand
Predictive demand refers to the practice of forecasting future customer demand based on historical data, market trends, and various other influencing factors. This definition encapsulates a range of methodologies that can be deployed to estimate demand for products and services. The methods often employ statistical techniques, machine learning algorithms, and qualitative assessments to produce actionable insights that guide business decisions.
Such forecasting can be vital across various sectors, notably retail and manufacturing, where understanding future demand increases operational efficiency. Accurate prediction allows businesses to minimize costs associated with overproduction or stockouts, enabling a more fluid response to market dynamics.
Historical Context and Evolution
The concept of predictive demand is not entirely new. Its roots can be traced back to traditional forecasting methods utilized in supply chain management. Historically, businesses relied upon basic statistical tools such as moving averages and seasonal adjustments to gauge demand.
As technology advanced, so did these methodologies. The introduction of computers in the 20th century allowed for more sophisticated statistical models and simulations. Today, the landscape has transformed with the emergence of big data analytics and machine learning.
Organizations can now harness vast amounts of relevant data, which leads to more accurate and timely predictions. Historical methods provided a foundation, but the evolution towards data-driven predictive demand models signifies a significant leap forward in business intelligence.
Importance of Predictive Demand
Predictive demand plays a pivotal role across multiple industries, influencing core areas such as supply chain management and strategic marketing. Understanding the mechanisms behind predictive demand is essential for organizations striving for efficiency, responsiveness, and competitive advantages in an increasingly complex market. Its significance stems not only from operational improvements but also from enhanced decision-making capabilities that are vital in today’s volatile landscape.
Impact on Supply Chain Management
The impact of predictive demand on supply chain management is profound. By accurately forecasting demand, companies can optimize their inventory levels, reducing unnecessary costs associated with overstocking or stockouts. This leads to improved cash flow and resource allocation.
- Efficiency in Production: Predictive demand allows for better planning in production schedules. Manufacturers can align their operations with anticipated consumer behavior, leading to more streamlined processes and reduced waste.
- Enhanced Logistics: With more reliable demand forecasts, logistics planning becomes more precise. Shipping routes can be optimized, and delivery times can be minimized, which enhances customer satisfaction.
Moreover, predictive analytics facilitate collaboration between suppliers and retailers. When both parties share insights into forthcoming demand trends, they can create a more resilient supply chain. The reliance on timely data transforms how organizations interact, fostering better partnerships.
Role in Strategic Marketing
In the realm of strategic marketing, predictive demand serves as a compass for guiding campaigns and promotional strategies. By analyzing consumer behavior patterns, businesses can tailor their marketing initiatives to align with what customers are likely to want.
- Targeted Campaigns: Predictions about future demand enable marketers to identify target audiences more effectively. This ensures that marketing efforts are focused on consumers whose interests align with upcoming product offerings.
- Product Development: Insights from predictive analytics can inform product development decisions. Understanding which products are likely to be in demand allows organizations to allocate resources efficiently.
Furthermore, businesses can monitor social media trends to refine their marketing strategies. Predictive demand tools can analyze discussions, reviews, and mentions across platforms like Facebook and Reddit, giving insights into consumer interests and preferences.
"Successful companies leverage predictive demand data to not only react to changes but also anticipate them, achieving a proactive stance in their marketing efforts."
Overall, the role of predictive demand in strategic marketing is instrumental in creating personalized experiences for customers, ultimately contributing to brand loyalty and sustained growth. By utilizing data-driven insights, organizations can navigate complexities effectively, adapting their strategies in real-time.
Methodologies for Forecasting Demand
Predictive demand relies heavily on methodologies that can provide accurate models for future trends. These methods help organizations anticipate fluctuations in market demand, ensuring efficient inventory management and optimal resource allocation. Various forecasting methodologies exist, including statistical methods and machine learning techniques, each offering unique benefits and considerations.
The choice of methodology directly impacts predictive accuracy and overall effectiveness in demand forecasting. For instance, while statistical methods such as time series analysis and regression analysis may have been more traditional approaches, machine learning techniques like neural networks and decision trees are gaining ground due to their ability to handle large datasets and uncover complex patterns.
Statistical Methods
Statistical methods are often regarded as the foundational tools for forecasting demand. These methods utilize historical data to identify trends and patterns that inform future projections. Among the statistical techniques, time series analysis and regression analysis stand out.
Time Series Analysis
Time series analysis focuses on analyzing data points collected or recorded at specific time intervals. This method emphasizes the temporal dimension of the data, allowing forecasters to identify trends, seasonal variations, and cyclical patterns.
A key characteristic of time series analysis is its ability to model data that is sensitive to time-related changes, making it a beneficial choice in industries where demand varies over time. The benefit of using time series analysis lies in its relatively straightforward application and interpretability. One unique feature is its reliance on historical performance as an indicator of future demand rather than external factors. However, it may not always accommodate sudden market shifts or irregular events, which could limit its accuracy.
Regression Analysis
Regression analysis, on the other hand, examines the relationship between a dependent variable and one or more independent variables. This analysis helps determine how various factors affect demand forecasts, enabling a more nuanced understanding of market drivers.
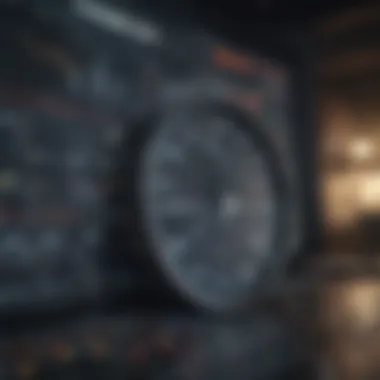
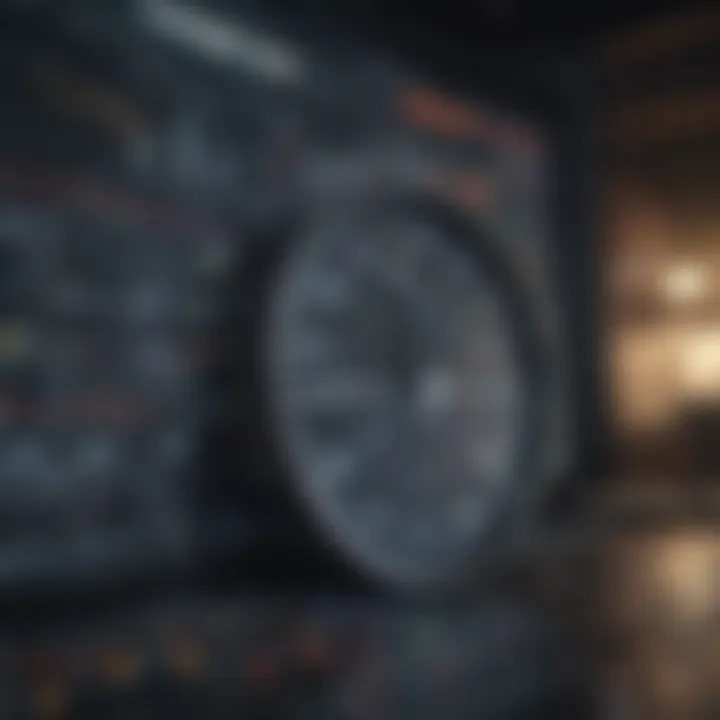
A prominent characteristic of regression analysis is its flexibility; it can incorporate multiple influencing variables, making it beneficial for businesses in diverse scenarios. Its unique feature includes the ability to quantify the impact of different variables on demand. However, the reliability of regression analysis can be compromised by issues like multicollinearity or omitted variable bias, requiring careful model selection and validation.
Machine Learning Techniques
Machine learning techniques have emerged as powerful tools for demand forecasting, especially as the volume and variety of data increase. These methods adaptively learn from data, improving predictive performance over time.
Neural Networks
Neural networks are sophisticated algorithms that simulate the human brain's processing capabilities, allowing for complex pattern recognition within data. This adaptability contributes significantly to its role in improving predictive demand strategies.
A key characteristic of neural networks is their ability to model non-linear relationships between inputs and outputs, making them a popular choice for forecasts that are influenced by multiple variables. Their unique feature is the capability to learn from vast quantities of historical data without being explicitly programmed for every scenario. However, they require significant computational resources and technical expertise for proper implementation and optimization.
Decision Trees
Decision trees represent another machine learning technique, utilizing a flowchart-like structure to visualize decision-making processes based on different variables. They can effectively capture the complexities of consumer behavior and preferences.
The key characteristic of decision trees is their transparency and ease of interpretation, making them a beneficial choice for stakeholders who seek to understand the rationale behind forecasts. Their unique feature is the capability to handle both categorical and continuous data, offering versatility in various applications. However, decision trees may suffer from overfitting if not properly managed, which can lead to poor performance on unseen data.
"Incorporating methodologies that combine both statistical and machine learning techniques can enhance the accuracy and reliability of demand forecasts."
Data Sources for Predictive Demand
Understanding data sources for predictive demand is essential for effective forecasting. This section explores the critical internal and external data sources that organizations can harness to enhance their predictive capabilities. By leveraging these sources, businesses can create accurate demand forecasts, improve inventory management, and tailor marketing strategies effectively.
Internal Data Collection
Sales Records
Sales records provide crucial insights into past performance and customer purchase behaviors. They contain detailed information such as sales volume, revenue, and product combinations. This data helps businesses identify trends and seasonality in sales, which can be invaluable for predicting future demand. The primary characteristic of sales records is their historical nature. They reflect actual transactions, which makes them a reliable basis for demand forecasting. The benefit of using sales records is that they allow for data-driven decision making. This ground truth can significantly improve demand accuracy in various sectors. However, a unique feature of sales records is their dependency on internal processes and structures. If sales are not consistently recorded or if there are discrepancies, the forecasts may be compromised. Therefore, while using sales records is advantageous, it does require diligence in data collection practices.
Inventory Levels
Inventory levels represent the quantities of stock available at a given time. This data source is significant as it directly impacts the ability to meet customer demand. By tracking inventory levels, businesses can identify trends in stock depletion and adjust their ordering processes accordingly. A key characteristic of inventory levels is their dynamic nature, reflecting changes in both supply and demand. This aspect makes them a vital component of predictive demand analysis. With reliable inventory data, organizations can prevent stockouts and overstock situations, thereby enhancing operational efficiency. The unique feature of inventory levels lies in their real-time documentation. However, if inventory tracking systems are flawed or inaccurate, it can lead to costly errors. Hence, while they serve as a powerful tool for demand forecasting, accuracy in inventory management is crucial.
External Data Utilization
Market Trends
Market trends encapsulate the broader movements in consumer spending and industry performance. They can include data on emerging technologies, economic indicators, and shifts in consumer preferences. By analyzing market trends, businesses can align their offerings with customers' evolving needs. The key characteristic of market trends is their foresight. They provide crucial insights that go beyond immediate sales data, allowing companies to anticipate changes in demand. This forward-looking information is highly beneficial for long-term strategic planning. A unique feature of market trends is their influence on consumer behavior. However, their analysis can be complex due to the volatility of market forces. Therefore, relying solely on market trends without considering internal data may lead to inaccurate predictions.
Consumer Behavior Data
Consumer behavior data captures insights into how individuals or groups select, buy, use, and dispose of products. This data can be collected from various touchpoints, including surveys, social media interactions, and web analytics. Understanding consumer behavior is crucial for creating personalized marketing strategies and improving customer satisfaction. The key characteristic of consumer behavior data is its granularity. It offers deep insights into customer preferences, enabling businesses to tailor their products and marketing efforts. This specificity makes it a beneficial source for enhancing demand forecasts. A unique feature of this data is its real-time capacity to capture shifts in consumer sentiment. Yet, there may be challenges such as data privacy concerns and the potential for biased responses, which can limit its effectiveness. Thus, while consumer behavior data is indispensable, it requires careful handling and analysis to yield accurate insights.
"Data-driven decisions are the foundation of effective predictive demand strategies. Understanding both internal and external data sources enhances forecasting accuracy and operational effectiveness."
By mastering these data sources, organizations gain a competitive advantage in predictive demand management. Each data source contributes uniquely, ensuring that forecasts are informed, actionable, and aligned with market realities.
Technological Innovations in Predictive Demand
In today's fast-paced market, technological innovations have become the backbone of predictive demand forecasting. These advancements not only enhance accuracy but also streamline processes to make businesses agile and responsive. Understanding these innovations is essential for anyone keen on diving deep into predictive demand methodologies.
Artificial Intelligence Applications
Artificial Intelligence (AI) plays a pivotal role in enhancing predictive demand capabilities. By utilizing complex algorithms, AI helps businesses recognize patterns in large datasets that human analysts may overlook. This leads to improved demand forecasting tailored to specific market dynamics.
AI applications can transform traditional forecasting in the following ways:
- Predictive Analytics: AI algorithms, such as those used in Google Analytics, analyze historical data to predict future trends. Businesses can confidently make inventory decisions.
- Real-time Processing: AI can process data instantly, allowing companies to respond to market changes as they happen. This is especially useful in industries with fluctuating demand.
- Customer Segmentation: AI can help businesses identify distinct consumer groups accurately. By analyzing purchasing behavior, businesses can tailor marketing strategies and product offerings.
However, the deployment of AI requires careful consideration. Organizations must ensure they have access to quality data. Furthermore, transparency in AI-driven decisions is crucial to maintain consumer trust.
Cloud Computing Impact
Cloud computing has revolutionized the way businesses manage their data for predictive demand. Its ability to provide scalable, flexible, and efficient services means organizations can store and analyze vast amounts of data without substantial upfront investments.
Key benefits of cloud computing in predictive demand include:
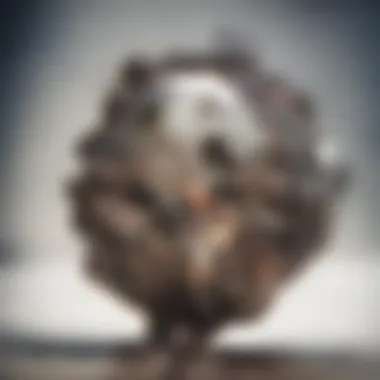
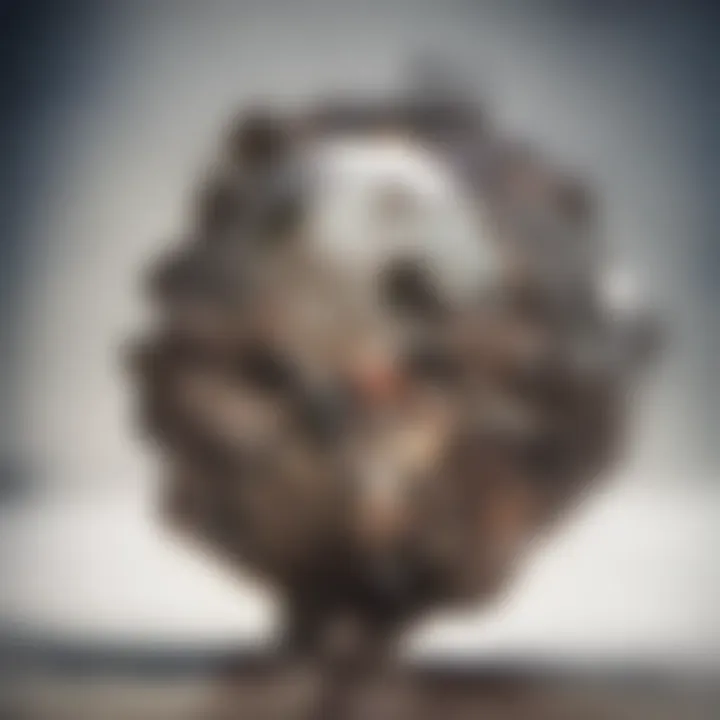
- Scalability: As business needs change, cloud services can quickly adjust to accommodate new data and increased processing needs, ensuring no interruption in predictive capabilities.
- Collaboration: Cloud platforms enable teams to access data and tools from anywhere, promoting collaboration. This is especially beneficial for businesses with distributed teams.
- Cost-Effectiveness: By opting for cloud solutions, businesses save on infrastructure costs. Pay-as-you-go models ensure that expenses align with actual usage, allowing for better financial planning.
The integration of cloud computing requires strategic planning. Organizations must consider cloud security and compliance to protect sensitive data. The future of predictive demand seems inherently tied to ongoing advancements in cloud technologies.
"Technological innovations, particularly AI and cloud computing, are not merely enhancements to predictive demand; they are essential for survival in a data-driven market."
In summary, technological innovations serve as catalysts for change in predictive demand. AI enhances analysis and accuracy while cloud computing provides the necessary infrastructure for seamless data management and processing. Understanding these elements is crucial for professionals aiming to leverage predictive demand insights effectively.
Consumer Behavior Analysis
Understanding consumer behavior is essential for businesses seeking to enhance their predictive demand capabilities. The behavior of consumers plays a critical role in shaping market trends and influences demand forecasting accuracy. By examining what drives customers’ purchasing decisions, businesses can better anticipate future demand and tailor their strategies accordingly.
Understanding Customer Needs
To effectively predict demand, it is vital to understand customer needs. This involves identifying not only what customers want but also the emotional and psychological factors that drive their choices. Insights can be obtained through different channels. For example, analyzing sales data helps reveal trends while direct feedback from customers provides qualitative insights. Understanding these needs helps firms adjust their inventory levels, promotional strategies, and product offerings.
- Key benefits of analyzing customer needs include:
- Improved product alignment with market demand
- Enhanced customer satisfaction and loyalty
- More effective advertising and marketing strategies
A business that grasps its customers’ needs can respond faster and more efficiently. This adaptability is crucial in today's rapidly changing market landscape.
Influence of Social Media Trends
Social media platforms have emerged as significant channels for understanding consumer behavior. This digital landscape offers real-time insights into trends, preferences, and sentiments. Marketers can leverage platforms like Facebook and Reddit to gauge consumer reaction to products and campaigns.
- Factors influenced by social media include:
- Viral marketing effects
- User-generated content driving engagement
- Immediate feedback loops that inform product adjustments
Social media not only shapes consumer perceptions but also generates data that can be utilized in predictive modeling, enhancing the overall effectiveness of demand forecasts.
Engaging with consumers through social media also fosters a community around a brand. By facilitating dialogue, businesses can stay ahead of market shifts and adapt their offerings in response to these insights.
Challenges in Predictive Demand
Predictive demand is a powerful tool in modern business strategy, yet it comes with its own set of challenges. Understanding these challenges is essential for researchers, students, and professionals alike. The ability to predict future demand accurately can transform supply chain management and marketing but requires overcoming several significant hurdles. The main challenges that businesses must navigate include data quality issues and changing market dynamics.
Data Quality Issues
Data quality is crucial for effective predictive demand models. The accuracy of predictions relies heavily on the data used in generating these forecasts. Poor data quality can lead to misleading insights, which can ultimately result in poor decision-making. There are several elements to consider regarding data quality:
- Completeness: Incomplete data sets can skew results. Businesses must ensure enough data is collected to form accurate predictions.
- Consistency: Data should remain consistent across different sources. Disparities can introduce errors in analysis.
- Timeliness: Data must be current. Outdated information can render forecasts irrelevant.
- Accuracy: Errors in data entry or processing can lead to wrong conclusions.
Ensuring high data quality involves a commitment to meticulous data management practices. Organizations should invest in training for staff involved in data entry and analysis. Utilizing tools that monitor data integrity may also prove beneficial. In some cases, companies may choose to collaborate with third-party data providers to ensure that the data they use for predictive analysis is robust and reliable.
Changing Market Dynamics
Another prominent challenge in predictive demand is the ever-evolving landscape of market dynamics. Factors such as economic fluctuations, shifts in consumer preferences, and emerging technologies can radically alter demand forecasts. Companies must stay vigilant and adapt their strategies accordingly. Consider the following:
- Economic Changes: Economic downturns or booms can rapidly shift consumer spending patterns. Predictive models must account for these fluctuations.
- Consumer Trends: Social media and cultural trends can shape what products are in demand. Businesses need to monitor these trends closely to adjust forecasts.
- Technological Advances: New technologies can introduce unexpected changes in market behavior. Companies should remain aware of these advancements when formulating demand models.
Maintaining flexibility within predictive models can alleviate some issues arising from changing market dynamics. Agile methodologies may allow organizations to update their forecasts regularly and respond to new information promptly. Collaboration across various teams—such as marketing, sales, and supply chain—can facilitate a holistic approach to predictive demand, ensuring that companies are prepared for whatever shifts may arise.
"Effective predictive demand forecasting requires a nuanced understanding of both data integrity and external market forces."
By addressing these challenges head-on, companies can achieve more accurate and actionable predictive insights. A focus on data quality and responsiveness to market dynamics will ultimately lead to improved decision-making and strategic planning.
Ethical Considerations
Understanding the ethical implications of predictive demand is vital in today’s data-driven landscape. As businesses increasingly rely on data analytics and machine learning for demand forecasting, they face significant ethical dilemmas. These considerations influence how data is collected, processed, and utilized. Failing to address these issues can not only affect company reputation but also undermine consumer trust.
Data Privacy Concerns
Data privacy is a pressing concern when it comes to predictive demand. Companies collect vast amounts of personal information to enhance their forecasting accuracy. This data often includes sensitive consumer details, such as purchase history and personal preferences. It is essential for businesses to prioritize the privacy of this data.
Organizations must comply with laws like the General Data Protection Regulation (GDPR) in the European Union. This regulation mandates transparency in how data is collected and allows users to request data deletion. Not adhering to these legal regulations can lead to severe penalties and loss of customer confidence.
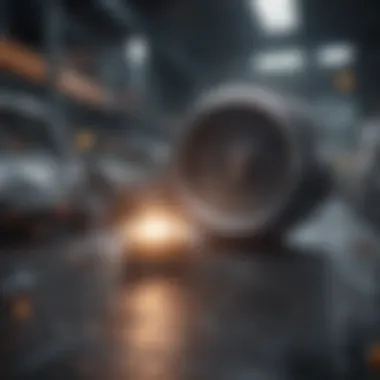
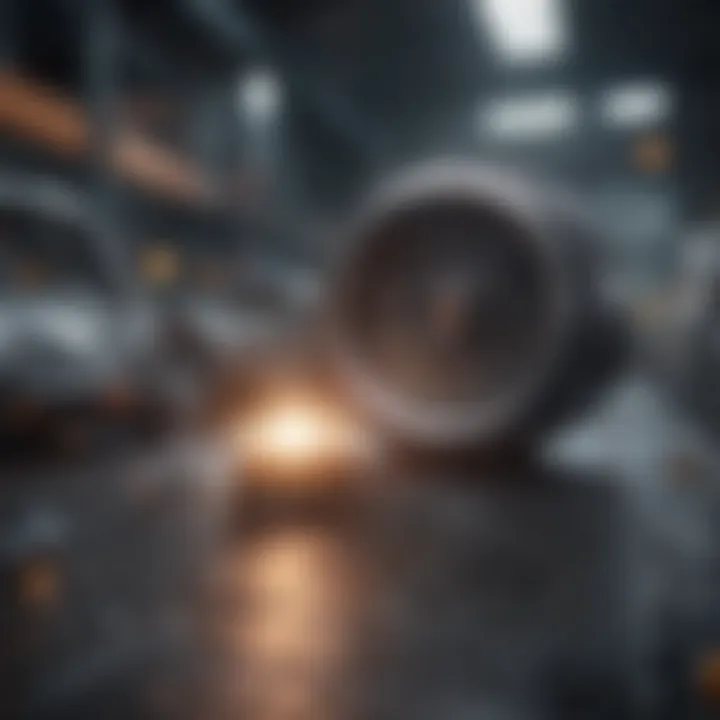
Moreover, ethical data collection practices include ensuring that consumers are well informed about their data usage. Businesses should implement clear data policies and offer opt-in mechanisms for consumers. Fostering an environment of trust will enhance rapport with customers, creating a more receptive audience for future marketing efforts.
Bias in Data Collection
Bias in data collection is another critical ethical consideration within predictive demand. If data is not collected or analyzed correctly, it can lead to skewed results that reinforce existing stereotypes. For instance, if a company relies predominantly on a narrow demographic for its predictive models, it may misrepresent the preferences and behaviors of broader consumer groups.
It is crucial to ensure data diversity. This can be achieved by including data from varied sources and demographic backgrounds. Companies should also regularly audit their data collection methods to identify and rectify biases. Acknowledging and addressing bias helps in forming fair and accurate predictive models, leading to better business decisions.
"The responsibility lies with organizations to ensure that their predictive analytics practices are transparent and ethical. Failure to do so can result in significant ramifications, both legally and reputationally."
In summary, ethical considerations in predictive demand are multifaceted. With growing scrutiny around how consumer data is handled, organizations must build frameworks that prioritize privacy and mitigate bias. Addressing these issues is not merely a compliance exercise; it fundamentally impacts the relationship between businesses and their customers.
Industry Applications of Predictive Demand
The applications of predictive demand extend far beyond theoretical frameworks. They are a fundamental element of decision-making in various industries. Understanding how predictive demand influences different sectors helps in crafting strategies that can lead to enhanced efficiency and profitability. By analyzing and anticipating consumer needs, businesses can optimize their operations significantly. The focus will be on sectors such as retail and healthcare, which inherently rely on accurate demand forecasting to sustain and grow.
Retail Sector Applications
In the retail sector, predictive demand plays a crucial role in inventory management and customer satisfaction. Retailers utilize forecasting to ensure they have the right amount of stock at the right time. This not only helps in meeting customer demands but also minimizes excess inventory, reducing holding costs.
Commonly used methods include:
- Sales Data Analysis: Retailers analyze historical sales data to predict future demand accurately. This helps in making informed stocking decisions.
- Promotional Planning: Understanding how promotions affect consumer purchasing behavior allows retailers to forecast demand more effectively during sales campaigns.
- Seasonal Trends: Recognizing seasonal patterns aids in optimizing inventory for different times of the year, such as holidays or back-to-school seasons.
The benefits of applying predictive demand in retail include improved operational efficiency, elevated customer satisfaction, and enhanced profit margins. When inventories align with actual demand, retailers not only save costs but also cultivate customer loyalty by minimizing stockouts.
Healthcare and Predictive Demand
In the healthcare industry, predictive demand is just as critical, if not more so. Accurate demand forecasting can greatly influence resource allocation and patient care. By anticipating patient needs, healthcare providers can streamline operations and optimize treatment efficacy.
Key uses of predictive demand in healthcare encompass:
- Patient Flow Management: Hospitals use predictive analytics to forecast patient admissions, leading to better staffing and resource management.
- Supply Chain Optimization: Predicting demand for medical supplies ensures that necessary equipment is available when needed, which is essential for patient care.
- Public Health Preparedness: During health crises, such as pandemics, being able to predict rising demand for certain treatments or services can greatly improve response times and health outcomes.
The implications of predictive demand in healthcare are far-reaching. Successful application can lead to reduced wait times, improved patient satisfaction, and optimized operational costs. With the increasing complexity of healthcare needs, leveraging predictive analytics is moving from an option to a necessity in managing health systems effectively.
Future Trends in Predictive Demand
The landscape of predictive demand is evolving rapidly, driven by advancements in technology and changes in consumer behavior. These trends are not merely theoretical; they have substantial implications for businesses striving to remain competitive. Understanding what lies ahead allows companies to harness predictive demand more effectively, improving operational efficiency while satisfying customer needs.
Advancements in Technology
Technological advancements are reshaping predictive demand methodologies. The rise of artificial intelligence and machine learning enhances the accuracy of demand forecasts. Algorithms can analyze vast amounts of data much faster than humans. Thus, machine learning techniques enable the development of richer models that predict demand patterns with greater precision.
For instance, real-time data processing technologies allow companies to react swiftly to demand changes. Businesses can implement systems that learn from new data, adapting forecasts almost instantaneously. This adaptability is crucial in industries like retail, where consumer preferences can shift rapidly. Additionally, cloud computing facilitates collaboration and data sharing, making it easier for various departments to access the information they need to make informed decisions.
Integration of Big Data
Big data's integration into predictive demand practices is another pivotal trend. Organizations can now utilize a myriad of data sources, revealing insights previously overlooked. By combining internal data such as sales records with external data like social media trends, businesses can gain a 360-degree view of demand drivers.
- Consumer Behavior: Analyzing online interactions and trends can predict future purchasing behaviors more reliably.
- Market Analysis: Businesses can evaluate market conditions dynamically, adjusting strategies based on actionable insights.
Moreover, data analytics tools allow for segmentation of customers, leading to more tailored marketing strategies. This can significantly enhance customer engagement and drive sales. As more data continues to flow, companies need robust systems to manage and analyze this information efficiently.
"The future of predictive demand relies on the ability to aggregate and analyze diverse data sets in real time, ensuring businesses stay ahead of market shifts."
Finale
The conclusion serves as a vital component of this article on predictive demand, tying together the various threads explored throughout. It reflects on the significant insights gained from examining predictive demand, emphasizing how these insights can influence decision-making in multiple sectors like supply chain management and marketing.
In summary, predictive demand plays a crucial role in aligning business strategies with consumer needs. The methodologies discussed, such as machine learning and statistical methods, equip organizations with the tools necessary to anticipate shifts in demand. Moreover, understanding data sources and technological innovations can provide a competitive edge in today’s fast-paced market environment.
A holistic view of predictive demand encourages organizations to invest in robust data analytics frameworks. This investment enables more accurate forecasting, resulting in improved resource allocation and enhanced customer satisfaction.
"Harnessing predictive demand not only streamlines operations but also fortifies strategic positioning in any industry."
The implications of this understanding extend beyond immediate benefits. Ethical considerations related to data privacy and potential biases in data collection must be managed prudently. Addressing these aspects is essential for fostering trust and ensuring long-term sustainability in predictive demand initiatives.
Summary of Key Insights
- Predictive demand is integral to forecasting consumer needs accurately.
- Employing advanced methodologies, such as machine learning and regression analysis, enhances the reliability of predictions.
- The importance of both internal and external data sources cannot be overstated; they provide foundational insights for accurate forecasting.
- Technological innovations like artificial intelligence and cloud computing significantly impact how organizations analyze and respond to demand signals.
- Ethical considerations surrounding data usage necessitate careful attention to maintain consumer trust and integrity in predictive practices.
Final Thoughts on Predictive Demand
Ultimately, predictive demand is more than just a tool; it is a strategic asset that can transform the way businesses operate. By prioritizing ethical considerations and advancing their analytical capabilities, companies can leverage predictive demand to not only meet but exceed consumer expectations.